autogenu-jupyter
autogenu-jupyter copied to clipboard
An automatic code generator for nonlinear model predictive control (NMPC) and the continuation/GMRES method (C/GMRES) based numerical solvers for NMPC
AutoGenU for Jupyter
Introduction
This project provides the continuation/GMRES method (C/GMRES method) based solvers for nonlinear model predictive control (NMPC) and an automatic code generator for NMPC, called AutoGenU.
The following C/GMRES based solvers are provided:
-
MultipleShootingCGMRESSolver
: The multiple shooting based C/GMRES method with condensing of the state and the Lagragne multipliers with respect to the state equation. -
SingleShootingCGMRESSolver
: The original C/GMRES method (single shooting).
Requirement
- C++17 (MinGW or MSYS and PATH to either are required for Windows users)
- CMake
- Python 3.8 or later, Jupyter Lab or Jupyter Notebook, SymPy, and collection (to generate
ocp.hpp
,main.cpp
, andCMakeLists.txt
byAutoGenU.ipynb
) - Python 3.8 or later, NumPy, Matplotlib, and seaborn (to plot simulation data on
AutoGenU.ipynb
) - ffmpeg (to generate animations in
pendubot.ipynb
,cartpole.ipynb
,hexacopter.ipynb
, andmobilerobot.ipynb
)
The python modules can be installed via
pip install -r requirements.txt
Usage
Submodules
Please confirm that you clone this repository as
git clone https://github.com/mayataka/autogenu-jupyter --recursive
Otherwise, please do the following command:
git submodule update --init --recursive
AutoGenU
AutoGenU.ipynb
generates following source files under your setting state equation, constraints, cost function, and parameters:
-
ocp.hpp
-
main.cpp
-
CMakeLists.txt
You can also build source files for numerical simulation, execute numerical simulation, and plot or save simulation result on AutoGenU.ipynb
.
C/GMRES based solvers of NMPC
The C/GMRES based solvers in src/solver
directory can be used independently of AutoGenU.ipynb
. You are then required the following files:
-
ocp.hpp
: write the optimal control problem (OCP) in your model
In addition to these files, you have to write CMakeLists.txt
to build source files.
Demos
Demos are presented in cartpole.ipynb
, pendubot.ipynb
, hexacopter.ipynb
, and mobilerobot.ipynb
. You can obtain the following simulation results jusy by runnig these .ipynb
files. The details of the each OCP formulations are described in each .ipynb
files.
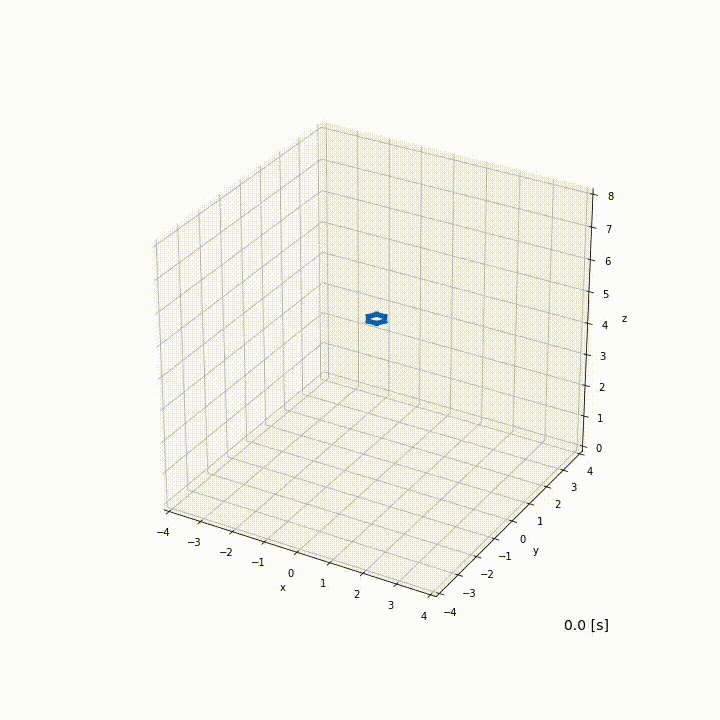
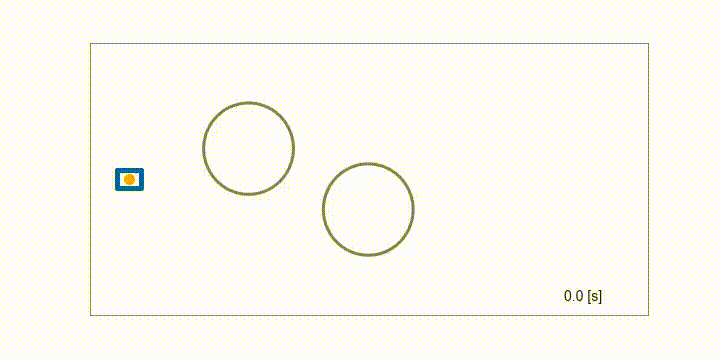
License
MIT
Citing autogenu-jupyter
We'd appriciate if you use cite the following conference paper:
@inproceedings{katayama2020autogenu,
title={Automatic code generation tool for nonlinear model predictive control with {J}upyter},
author={Sotaro Katayama and Toshiyuki Ohtsuka},
booktitle={{The 21st IFAC World Congress 2020}},
pages={7033-7040},
year={2020}}
References
- T. Ohtsuka A continuation/GMRES method for fast computation of nonlinear receding horizon control, Automatica, Vol. 40, No. 4, pp. 563-574 (2004)
- C. T. Kelly, Iterative methods for linear and nonlinear equations, Frontiers in Apllied Mathematics, SIAM (1995)
- Y. Shimizu, T. Ohtsuka, M. Diehl, A real‐time algorithm for nonlinear receding horizon control using multiple shooting and continuation/Krylov method, International Journal of Robust and Nonlinear Control, Vol. 19, No. 8, pp. 919-936 (2008)