xiaosi
xiaosi
> 第一个问题:我之前用多卡并行训练了一个512x512分辨率的模型,我只测了客观指标,还没有看具体的结果,麻烦您帮我也试一下哈。模型链接在这https://github.com/junleen/RainNet#update > > 第二个问题:这个case有点意思,局部靠近边界的地方产生了一些乖乖的颜色,我后面可能得具体找找原因,感谢您的提问。 感谢您的及时回复, 1.我测试的这张图是HCOCO数据集的c10123_2152925_1.jpg图片,您可以自己测试一下,看看是否和我一样的效果 2.对于新的模型,除了修改模型,对于test.py 文件 testdata = TestDataset(foreground_paths=comp_path, mask_paths=mask_path, background_paths=real_path, load_size=256),这个load_size 设置有什么影响吗,我尝试我这里最高设置1024,输出为1024。 对于原始的load_size=256:得到这个结果 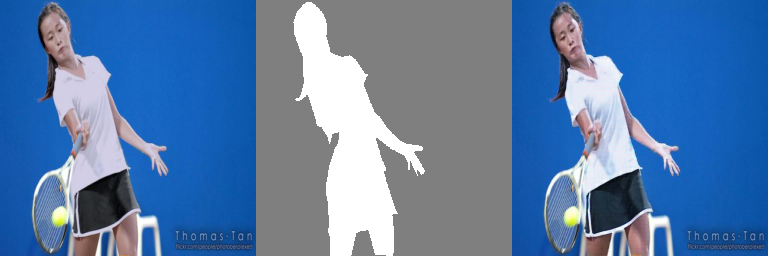 我修改load_size=1024,得到这个结果 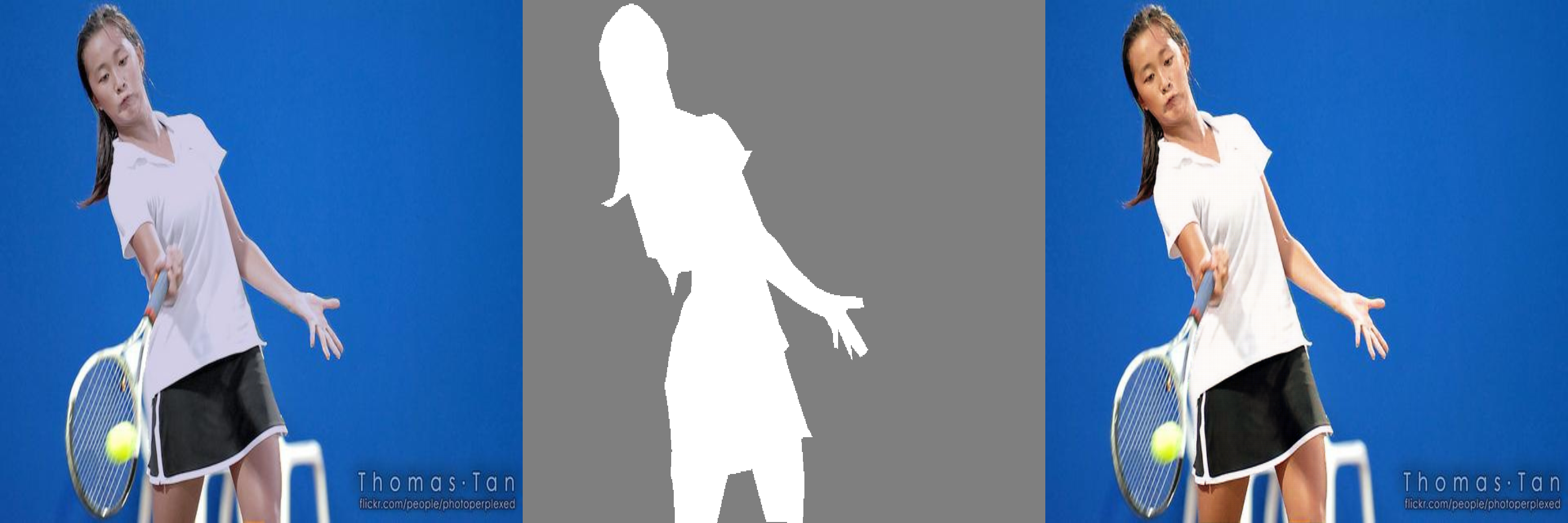 您看一下有什么区别
> 一般是在多少分辨率训练的,就在多少分辨测试。如果是512分辨率的话,用256测试可能不太行。您这里尝试的测试结果,在1024分辨率感觉还挺好的呀。 1.是的,我也觉得效果很好,那我如何才能测试更大分辨率的图像呢,比如2k、4k之类的图像 2.对于咱们这个实现和谷歌(https://github.com/augmentedperception/total_relighting)的这个实现,本质一样吗,都是处理合成之后的效果 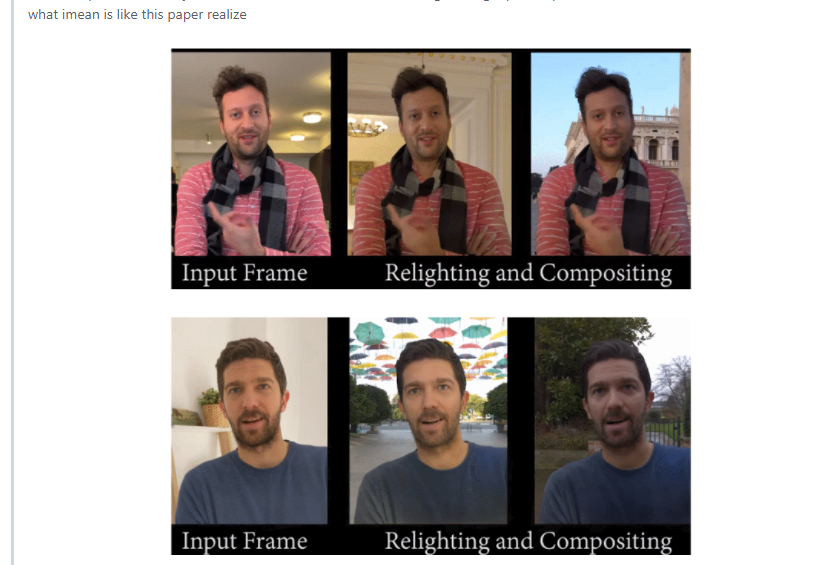 3.这个项目,相较于其他的两个项目,有什么不同之处呢 https://github.com/bcmi/BargainNet-Image-Harmonization https://github.com/bcmi/Image-Harmonization-Dataset-iHarmony4
> 3\. 的论文方法嘛 1感谢您的回复,我能输出分辨率不一样的图像吗,比如1920*1080 ,还是说只能输出2的n次方数值(256*256、512*512、1024*1024、2048*4028...) 2. 我用新的512模型测试一张图像, 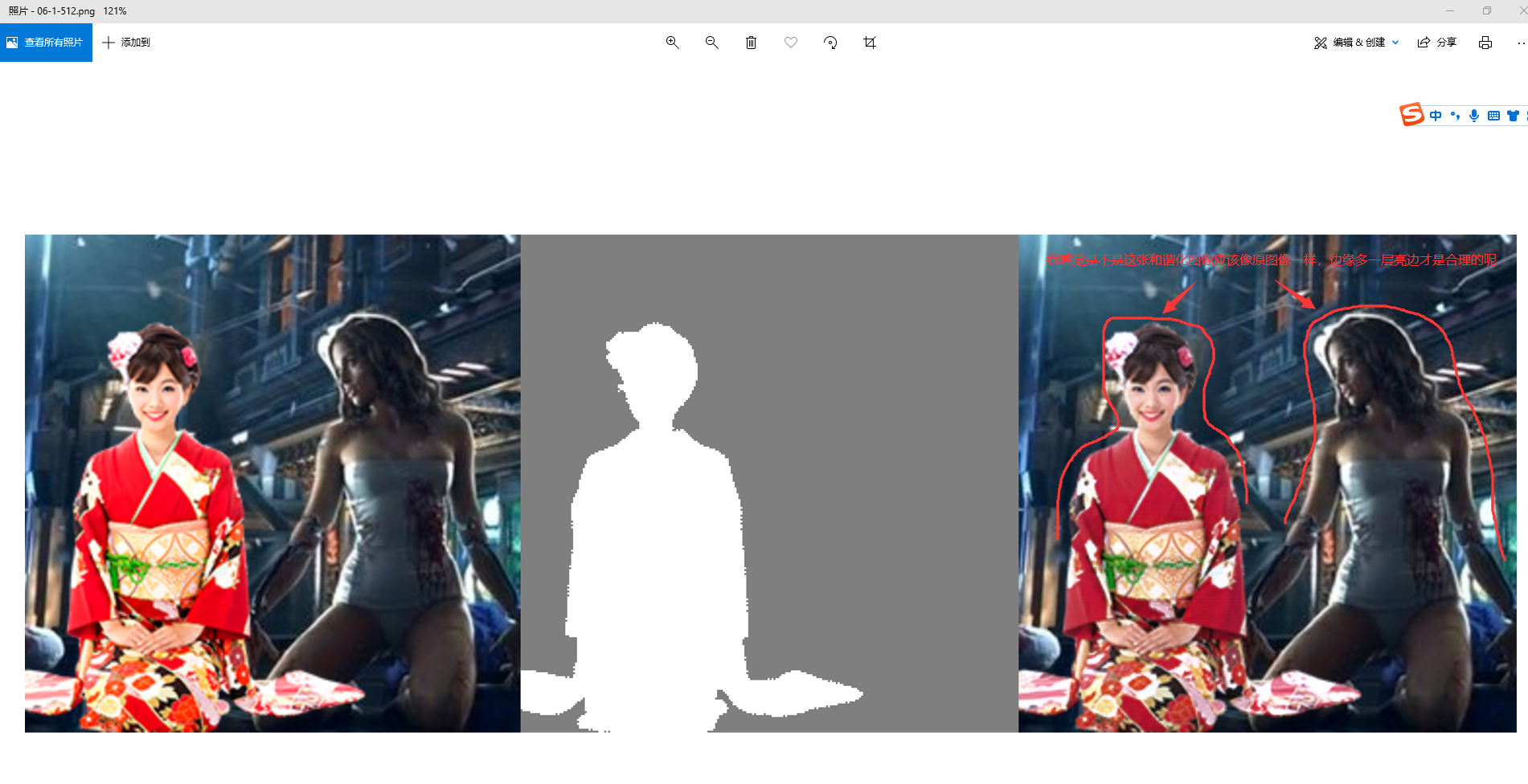
> 谢谢提问! > > 1. 2K、4K图像的话,可能得找轻量化的卷积模块,再加上我们的RAIN模块,现有的设计往高分辨率做容易消耗比较多的显存 > 2. 跟Google的论文方法和效果不是一样的。他的效果也还挺好的,但是偏向于环境光照的特征,可以跟进研究一下。事实上我们的目的是一样的,make the composite image more photo-realistic > 3. 这个不就是不同的论文方法嘛 您好,我想请教一下, 1.对于图像是不是需要固定特殊的尺寸,比如2的n次幂,能不能实现任意图像的处理,无需进行resize处理 2. 是不是对于任意的提取特征的网络作为骨架都是可行的,比如现在常用的ResNet 以及MobileNetv3 ,在这些特征图之间加上Rain模块可以实现 3. 对于速度上有什么好的优化呢,我测试的一张图像只进行处理最快大概需要1.2-1.3s,能不能实现实时(30fps) 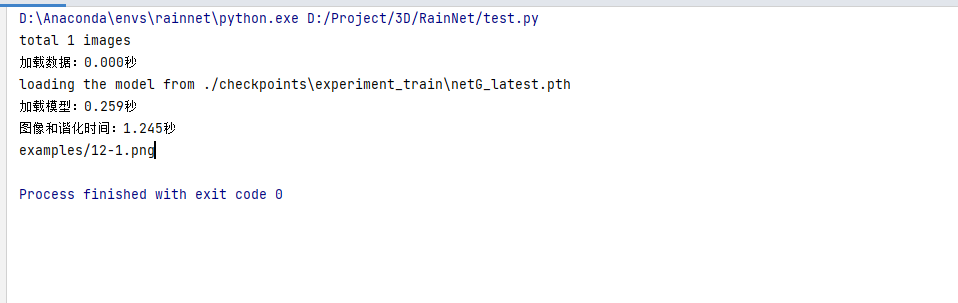
> Hi, > > First, some basic information about my setup which may be relevant. I am running Pyton 3.8.1, which was installed as part of Anaconda. I am using...
> You may try to use the torch.nn.functional.grid_sample to replace the trilinear function if you have difficulty in building up the cuda implementation. Please refer to #14 for more information....
> Sorry, what do you mean about too accurate? You can get your jpg image as model input and prepare your ground truth mask as binarized image with either pixel...
> I've create an onnx model from the pytorch model from this repo: https://github.com/ajbrock/BigGAN-PyTorch.git > > I can use the model in python, without pytorch only using onnx. > >...
> #15 看这个讨论,可能是由于cpu数据传输的原因 您好,大佬想请教一下,作者原文RVM 在4k视频可以达到76fps,我想在3090上达到最快速度推理,基于咱们这个库如何改进呢,这里推理包括数据加载方式、数据预处理以及模型推理得到输出值alpha、fgr,不包含后续合成
> 大佬,目的是使用C++推理,1.使用TensorRT会不会增加推理速度呢,2.我看你回复其他人问题,有提到rxi是在cpu操作的, 3.视频加载使用什么能提速?opencv GPU版本还是ffmpeg gpu?,预处理是否必须在cpu下才能操作。