External-Attention-pytorch
External-Attention-pytorch copied to clipboard
🍀 Pytorch implementation of various Attention Mechanisms, MLP, Re-parameter, Convolution, which is helpful to further understand papers.⭐⭐⭐
add shuffletransformer module
if __name__ == '__main__': input=torch.randn(3,256,7,7) danet=DAModule(d_model=256,kernel_size=3,H=7,W=7) print(danet(input).shape) input=torch.randn(3,256,7,7) when i change input to 3,256,128,128),wrong why?
I think, there are some problem with CondConv implementation because it's almost the same as for DynamicConv and differs from the original paper's architecture (according to the paper, it should...
WeightedPermuteMLP 中采用了几个全连接层Linear,具体代码位置在ViP.py中的21-23行 ```python self.mlp_c=nn.Linear(dim,dim,bias=qkv_bias) self.mlp_h=nn.Linear(dim,dim,bias=qkv_bias) self.mlp_w=nn.Linear(dim,dim,bias=qkv_bias) ``` 这几个线性层的输入输出通道数都是dim,即输入输出的通道数不变 在forward时,除了mlp_c是直接输入了x没有什么问题 ```python def forward(self,x) : B,H,W,C=x.shape c_embed=self.mlp_c(x) S=C//self.seg_dim h_embed=x.reshape(B,H,W,self.seg_dim,S).permute(0,3,2,1,4).reshape(B,self.seg_dim,W,H*S) h_embed=self.mlp_h(h_embed).reshape(B,self.seg_dim,W,H,S).permute(0,3,2,1,4).reshape(B,H,W,C) w_embed=x.reshape(B,H,W,self.seg_dim,S).permute(0,3,1,2,4).reshape(B,self.seg_dim,H,W*S) w_embed=self.mlp_w(w_embed).reshape(B,self.seg_dim,H,W,S).permute(0,2,3,1,4).reshape(B,H,W,C) weight=(c_embed+h_embed+w_embed).permute(0,3,1,2).flatten(2).mean(2) weight=self.reweighting(weight).reshape(B,C,3).permute(2,0,1).softmax(0).unsqueeze(2).unsqueeze(2) x=c_embed*weight[0]+w_embed*weight[1]+h_embed*weight[2] x=self.proj_drop(self.proj(x)) ``` 其他的两个线性层在使用时都有问题 可以看到这一步 ```python h_embed=x.reshape(B,H,W,self.seg_dim,S).permute(0,3,2,1,4).reshape(B,self.seg_dim,W,H*S) ```...
Hello, I think Criss-Cross Attention & Axial Attention are also the commonly used attention mechanisms.
The link seems wrong, it is the same to ‘Coordinate Attention for Efficient Mobile Network Design’
https://github.com/xmu-xiaoma666/External-Attention-pytorch/blob/2f80b03ef1cdd835d4a2d21eff6f8b3534e5d601/model/attention/CoAtNet.py#L21 Correct me, if I am wrong but isn't MLP usually a collection of fully-connected layers and not convolution layers?
paper:Fully Attentional Network for Semantic Segmentation 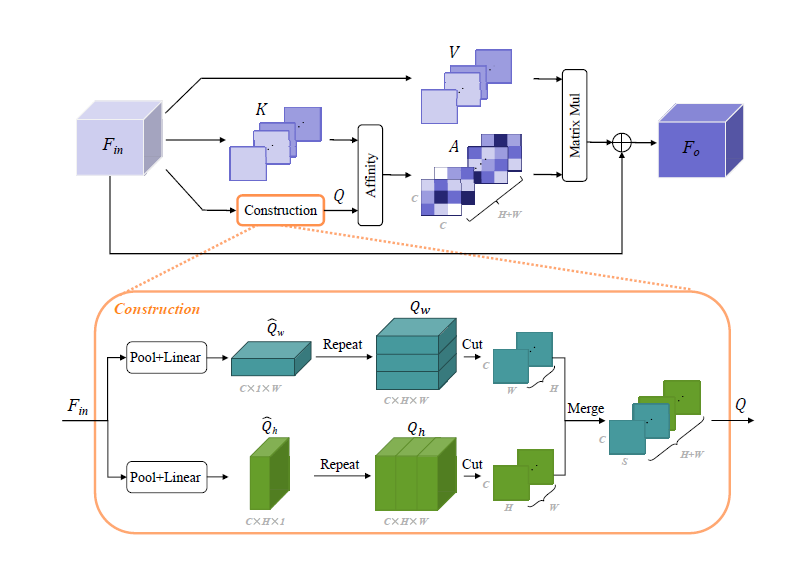