yolov5
yolov5 copied to clipboard
varying Scale of objects in detection
Search before asking
- [X] I have searched the YOLOv5 issues and discussions and found no similar questions.
Question
Hello ,
My dataset has objects ranging from very tiny to 50X bigger , I see that the hyperparameter config file has a parameter "scale" . would like to know if playing around with it would yield me better results ?
Note : I tried with default 0.5 and there are quite a lot of objects in FN .
Thanks
Additional
No response
@Akhp888 👋 Hello! Thanks for asking about image augmentation. YOLOv5 🚀 applies online imagespace and colorspace augmentations in the trainloader (but not the val_loader) to present a new and unique augmented Mosaic (original image + 3 random images) each time an image is loaded for training. Images are never presented twice in the same way.
Augmentation Hyperparameters
The hyperparameters used to define these augmentations are in your hyperparameter file (default data/hyp.scratch.yaml
) defined when training:
python train.py --hyp hyp.scratch-low.yaml
https://github.com/ultralytics/yolov5/blob/b94b59e199047aa8bf2cdd4401ae9f5f42b929e6/data/hyps/hyp.scratch-low.yaml#L6-L34
Augmentation Previews
You can view the effect of your augmentation policy in your train_batch*.jpg images once training starts. These images will be in your train logging directory, typically yolov5/runs/train/exp
:
train_batch0.jpg
shows train batch 0 mosaics and labels:
YOLOv5 Albumentations Integration
YOLOv5 🚀 is now fully integrated with Albumentations, a popular open-source image augmentation package. Now you can train the world's best Vision AI models even better with custom Albumentations 😃!
PR https://github.com/ultralytics/yolov5/pull/3882 implements this integration, which will automatically apply Albumentations transforms during YOLOv5 training if albumentations>=1.0.3
is installed in your environment. See https://github.com/ultralytics/yolov5/pull/3882 for full details.
Example train_batch0.jpg
on COCO128 dataset with Blur, MedianBlur and ToGray. See the YOLOv5 Notebooks to reproduce:
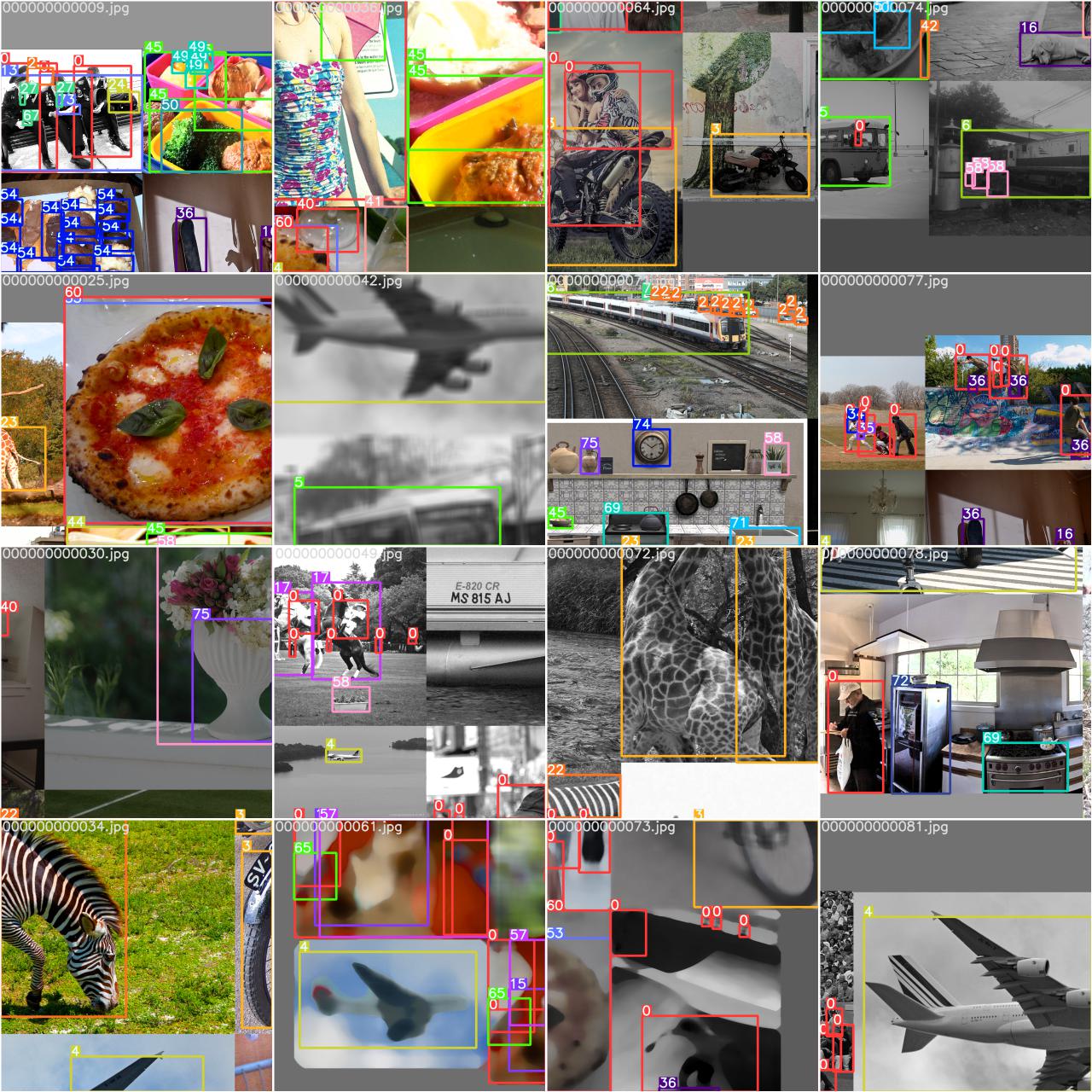
Good luck 🍀 and let us know if you have any other questions!
@glenn-jocher Thanks ,
so enabling Mosaic in hyperparameters adds objects of varying sizes ? Then i am curious to know what the "scale" does ? if there is any documentation for functionality of each variables in hyperparameter , would be great to know where i can find it .
https://medium.com/augmented-startups/how-hyperparameters-of-yolov5-works-ec4d25f311a2 These are the biggest ones
👋 Hello, this issue has been automatically marked as stale because it has not had recent activity. Please note it will be closed if no further activity occurs.
Access additional YOLOv5 🚀 resources:
- Wiki – https://github.com/ultralytics/yolov5/wiki
- Tutorials – https://docs.ultralytics.com/yolov5
- Docs – https://docs.ultralytics.com
Access additional Ultralytics ⚡ resources:
- Ultralytics HUB – https://ultralytics.com/hub
- Vision API – https://ultralytics.com/yolov5
- About Us – https://ultralytics.com/about
- Join Our Team – https://ultralytics.com/work
- Contact Us – https://ultralytics.com/contact
Feel free to inform us of any other issues you discover or feature requests that come to mind in the future. Pull Requests (PRs) are also always welcomed!
Thank you for your contributions to YOLOv5 🚀 and Vision AI ⭐!
@MartinPedersenpp thanks for sharing the link! The article seems to be a helpful resource for understanding YOLOv5 hyperparameters. As for your question about the "scale" hyperparameter, it controls the jitter of the image and grid sizes during training to allow some variation in object sizes and positions. You might tweak the scale for datasets with widely varying object sizes to permit better model understanding.
For all hyperparameters, including their functionality, you can refer to the official Ultralytics YOLOv5 documentation https://docs.ultralytics.com/yolov5/training-hyperrparameters. It comprehensively explains each hyperparameter and their effects during training.
Please let me know if you have any other questions or need further assistance!