yolov5
yolov5 copied to clipboard
RuntimeError: CUDA error: CUBLAS_STATUS_EXECUTION_FAILED
Search before asking
- [X] I have searched the YOLOv5 issues and discussions and found no similar questions.
Question
YOLOV5 + torch1.8.0 +cuda10.2+GTX1650 OS:Windows 10 python 3.9
(mmdeploy) D:\widows_mm\yolov5-7.0>python segment/train.py --weights yolov5n-seg.pt --img 640 --batch-size 2 --data data.yaml segment\train: weights=yolov5n-seg.pt, cfg=, data=data.yaml, hyp=data\hyps\hyp.scratch-low.yaml, epochs=100, batch_size=2, imgsz=640, rect=False, resume=False, nosave=False, noval=False, noautoanchor=False, noplots=False, evolve=None, bucket=, cache=None, image_weights=False, device=, multi_scale=False, single_cls=False, optimizer=SGD, sync_bn=False, workers=8, project=runs\train-seg, name=exp, exist_ok=False, quad=False, cos_lr=False, label_smoothing=0.0, patience=100, freeze=[0], save_period=-1, seed=0, local_rank=-1, mask_ratio=4, no_overlap=False github: skipping check (not a git repository), for updates see https://github.com/ultralytics/yolov5 YOLOv5 2022-11-22 Python-3.9.12 torch-1.8.0 CUDA:0 (NVIDIA GeForce GTX 1650, 4096MiB)
hyperparameters: lr0=0.01, lrf=0.01, momentum=0.937, weight_decay=0.0005, warmup_epochs=3.0, warmup_momentum=0.8, warmup_bias_lr=0.1, box=0.05, cls=0.5, cls_pw=1.0, obj=1.0, obj_pw=1.0, iou_t=0.2, anchor_t=4.0, fl_gamma=0.0, hsv_h=0.015, hsv_s=0.7, hsv_v=0.4, degrees=0.0, translate=0.1, scale=0.5, shear=0.0, perspective=0.0, flipud=0.0, fliplr=0.5, mosaic=1.0, mixup=0.0, copy_paste=0.0 TensorBoard: Start with 'tensorboard --logdir runs\train-seg', view at http://localhost:6006/ Overriding model.yaml nc=80 with nc=2
from n params module arguments
0 -1 1 1760 models.common.Conv [3, 16, 6, 2, 2] 1 -1 1 4672 models.common.Conv [16, 32, 3, 2] 2 -1 1 4800 models.common.C3 [32, 32, 1] 3 -1 1 18560 models.common.Conv [32, 64, 3, 2] 4 -1 2 29184 models.common.C3 [64, 64, 2] 5 -1 1 73984 models.common.Conv [64, 128, 3, 2] 6 -1 3 156928 models.common.C3 [128, 128, 3] 7 -1 1 295424 models.common.Conv [128, 256, 3, 2] 8 -1 1 296448 models.common.C3 [256, 256, 1] 9 -1 1 164608 models.common.SPPF [256, 256, 5] 10 -1 1 33024 models.common.Conv [256, 128, 1, 1] 11 -1 1 0 torch.nn.modules.upsampling.Upsample [None, 2, 'nearest'] 12 [-1, 6] 1 0 models.common.Concat [1] 13 -1 1 90880 models.common.C3 [256, 128, 1, False] 14 -1 1 8320 models.common.Conv [128, 64, 1, 1] 15 -1 1 0 torch.nn.modules.upsampling.Upsample [None, 2, 'nearest'] 16 [-1, 4] 1 0 models.common.Concat [1] 17 -1 1 22912 models.common.C3 [128, 64, 1, False] 18 -1 1 36992 models.common.Conv [64, 64, 3, 2] 19 [-1, 14] 1 0 models.common.Concat [1] 20 -1 1 74496 models.common.C3 [128, 128, 1, False] 21 -1 1 147712 models.common.Conv [128, 128, 3, 2] 22 [-1, 10] 1 0 models.common.Concat [1] 23 -1 1 296448 models.common.C3 [256, 256, 1, False] 24 [17, 20, 23] 1 128863 models.yolo.Segment [2, [[10, 13, 16, 30, 33, 23], [30, 61, 62, 45, 59, 119], [116, 90, 156, 198, 373, 326]], 32, 64, [64, 128, 256]] Model summary: 225 layers, 1886015 parameters, 1886015 gradients, 6.9 GFLOPs
Transferred 361/367 items from yolov5n-seg.pt AMP: checks passed optimizer: SGD(lr=0.01) with parameter groups 60 weight(decay=0.0), 63 weight(decay=0.0005), 63 bias train: Scanning D:\widows_mm\yolov5-7.0\labelmedata\json2yolo-master\new_dir_shuang\labels\train2017... 1000 images, 0 train: WARNING Cache directory D:\widows_mm\yolov5-7.0\labelmedata\json2yolo-master\new_dir_shuang\labels is not writeable: [WinError 183] : 'D:\widows_mm\yolov5-7.0\labelmedata\json2yolo-master\new_dir_shuang\labels\train2017.cache.npy' -> 'D:\widows_mm\yolov5-7.0\labelmedata\json2yolo-master\new_dir_shuang\labels\train2017.cache' val: Scanning D:\widows_mm\yolov5-7.0\labelmedata\json2yolo-master\new_dir_shuang\labels\train2017... 1000 images, 0 ba val: WARNING Cache directory D:\widows_mm\yolov5-7.0\labelmedata\json2yolo-master\new_dir_shuang\labels is not writeable: [WinError 183] : 'D:\widows_mm\yolov5-7.0\labelmedata\json2yolo-master\new_dir_shuang\labels\train2017.cache.npy' -> 'D:\widows_mm\yolov5-7.0\labelmedata\json2yolo-master\new_dir_shuang\labels\train2017.cache'
AutoAnchor: 5.55 anchors/target, 1.000 Best Possible Recall (BPR). Current anchors are a good fit to dataset Plotting labels to runs\train-seg\exp3\labels.jpg... Image sizes 640 train, 640 val Using 2 dataloader workers Logging results to runs\train-seg\exp3 Starting training for 100 epochs...
Epoch GPU_mem box_loss seg_loss obj_loss cls_loss Instances Size
0%| | 0/500 00:00
Traceback (most recent call last):
File "D:\widows_mm\yolov5-7.0\segment\train.py", line 658, in cublasGemmEx( handle, opa, opb, m, n, k, &falpha, a, CUDA_R_16F, lda, b, CUDA_R_16F, ldb, &fbeta, c, CUDA_R_16F, ldc, CUDA_R_32F, CUBLAS_GEMM_DFALT_TENSOR_OP)
Hello, when I was training my own dataset, I reported an error when calculating mask loss. Is it related to @? The environment can be tested
Additional
No response
π Hello @jiaqizhang123-stack, thank you for your interest in YOLOv5 π! Please visit our βοΈ Tutorials to get started, where you can find quickstart guides for simple tasks like Custom Data Training all the way to advanced concepts like Hyperparameter Evolution.
If this is a π Bug Report, please provide screenshots and minimum viable code to reproduce your issue, otherwise we can not help you.
If this is a custom training β Question, please provide as much information as possible, including dataset images, training logs, screenshots, and a public link to online W&B logging if available.
For business inquiries or professional support requests please visit https://ultralytics.com or email [email protected].
Requirements
Python>=3.7.0 with all requirements.txt installed including PyTorch>=1.7. To get started:
git clone https://github.com/ultralytics/yolov5 # clone
cd yolov5
pip install -r requirements.txt # install
Environments
YOLOv5 may be run in any of the following up-to-date verified environments (with all dependencies including CUDA/CUDNN, Python and PyTorch preinstalled):
-
Notebooks with free GPU:
- Google Cloud Deep Learning VM. See GCP Quickstart Guide
- Amazon Deep Learning AMI. See AWS Quickstart Guide
-
Docker Image. See Docker Quickstart Guide
Status
If this badge is green, all YOLOv5 GitHub Actions Continuous Integration (CI) tests are currently passing. CI tests verify correct operation of YOLOv5 training, validation, inference, export and benchmarks on MacOS, Windows, and Ubuntu every 24 hours and on every commit.
@jiaqizhang123-stack π Hello! Thanks for asking about CUDA issues. You may simply be out of memory.
YOLOv5 π can be trained on CPU, single-GPU, or multi-GPU. When training on GPU it is important to keep your batch-size small enough that you do not use all of your GPU memory, otherwise you will see a CUDA Out Of Memory (OOM) Error and your training will crash. You can observe your CUDA memory utilization using either the nvidia-smi
command or by viewing your console output:

CUDA Out of Memory Solutions
If you encounter a CUDA OOM error, the steps you can take to reduce your memory usage are:
- Reduce
--batch-size
- Reduce
--img-size
- Reduce model size, i.e. from YOLOv5x -> YOLOv5l -> YOLOv5m -> YOLOv5s > YOLOv5n
- Train with multi-GPU at the same
--batch-size
- Upgrade your hardware to a larger GPU
- Train on free GPU backends with up to 16GB of CUDA memory:
AutoBatch
You can use YOLOv5 AutoBatch (NEW) to find the best batch size for your training by passing --batch-size -1
. AutoBatch will solve for a 90% CUDA memory-utilization batch-size given your training settings. AutoBatch is experimental, and only works for Single-GPU training. It may not work on all systems, and is not recommended for production use.
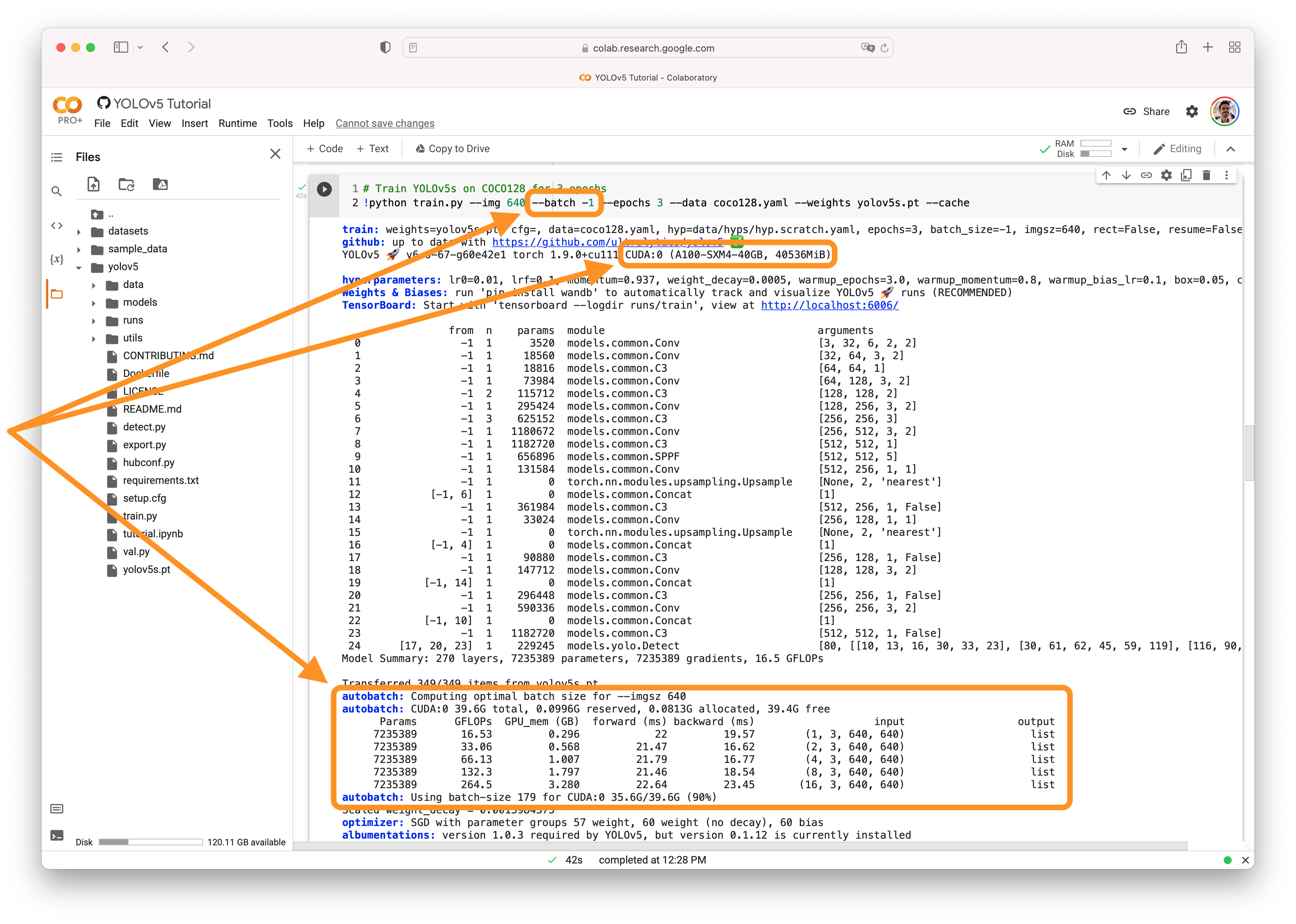
Good luck π and let us know if you have any other questions!
Hello, my training model is yolov5n seg, and the above error will also occur when the batch size is 1. I think this error has nothing to do with the back size, because it is an error when calculating the loss of a single image. When calculating a large matrix, the pred and proto have large dimensions, leading to cuda deficiency,
And when I replace βpred_mask = (pred @ proto.view(self.nm, -1)).view(-1, *proto.shape[1:])β with "pred_mask = torch.tensor(np.matmul(pred.cpu().detach().numpy(), proto.view(self.nm, -1).cpu().detach().numpy())).cuda().view(-1, *proto.shape[1:])", there will be no error.
At present, the GPU resources are sufficient, but an error occurs when calculating the matrix multiplication. Is this a bug in the GPU or is it caused by something? Thank you for your answer
@jiaqizhang123-stack there's no bug in the code, it's likely you are simply out of CUDA memory and this may be the highest-memory bottleneck that first trips an error. All Segmentation models were of course trained with GPUs without issue.
Is it necessary to train on a larger GPU? This code cannot train on a smaller GPU
@jiaqizhang123-stack yes I think you might need a larger GPU, or just to reduce memory usage using some of the tips above like smaller --imgsz.
I don't know if your fix will work as torch won't be able to calculate gradients after your .detach() and numpy ops.
OK, thank you very much
π Hello, this issue has been automatically marked as stale because it has not had recent activity. Please note it will be closed if no further activity occurs.
Access additional YOLOv5 π resources:
- Wiki β https://github.com/ultralytics/yolov5/wiki
- Tutorials β https://docs.ultralytics.com/yolov5
- Docs β https://docs.ultralytics.com
Access additional Ultralytics β‘ resources:
- Ultralytics HUB β https://ultralytics.com/hub
- Vision API β https://ultralytics.com/yolov5
- About Us β https://ultralytics.com/about
- Join Our Team β https://ultralytics.com/work
- Contact Us β https://ultralytics.com/contact
Feel free to inform us of any other issues you discover or feature requests that come to mind in the future. Pull Requests (PRs) are also always welcomed!
Thank you for your contributions to YOLOv5 π and Vision AI β!
@jiaqizhang123-stack you're very welcome! If you have any further questions or need assistance, feel free to ask. Good luck with your training!