TensorDiffEq
TensorDiffEq copied to clipboard
2D Wave Equation
Thank you for the great contribution!
I'm trying to extend the 1D example problems to 2D, but I want to make sure my changes are in the correct place:
- Dimension variables. I changed them like so:
Domain = DomainND(["x", "y", "t"], time_var='t')
Domain.add("x", [0.0, 5.0], 100) Domain.add("y", [0.0, 5.0], 100) Domain.add("t", [0.0, 5.0], 100)
- My IC is zero, but for the BCs I'm not sure how to define the left and right borders, please let me know if my implementation is correct:
def func_ic(x,y):
return 0
init = IC(Domain, [func_ic], var=[['x','y']])
upper_x = dirichletBC(Domain, val=0.0, var='x', target="upper")
lower_x = dirichletBC(Domain, val=0.0, var='x', target="lower")
upper_y = dirichletBC(Domain, val=0.0, var='y', target="upper")
lower_y = dirichletBC(Domain, val=0.0, var='y', target="lower")
BCs = [init, upper_x, lower_x, upper_y, lower_y]
All of my BCs and ICs are zero. And my equation has a (forcing) time-dependent source term as such:
def f_model(u_model, x, y, t):
c = tf.constant(1, dtype = tf.float32)
Amp = tf.constant(2, dtype = tf.float32)
freq = tf.constant(1, dtype = tf.float32)
sigma = tf.constant(0.2, dtype = tf.float32)
source_x = tf.constant(0.5, dtype = tf.float32)
source_y = tf.constant(2.5, dtype = tf.float32)
GP = Amp * tf.exp(-0.5*( ((x-source_x)/sigma)**2 + ((y-source_y)/sigma)**2 ))
S = GP * tf.sin( 2 * tf.constant(math.pi) * freq * t )
u = u_model(tf.concat([x,y,t], 1))
u_x = tf.gradients(u,x)
u_xx = tf.gradients(u_x, x)
u_y = tf.gradients(u,y)
u_yy = tf.gradients(u_y, y)
u_t = tf.gradients(u,t)
u_tt = tf.gradients(u_t,t)
f_u = u_xx + u_yy - (1/c**2) * u_tt + S
return f_u
Please advise.
Looking forward to your reply!
An update on this issue:
By running the previous changes I got these results. Any suggestions on how to improve the accuracy of this problem?
I also tried running the 1D wave equation with a source term S(x,t)
def f_model(u_model, x, t):
c = tf.constant(1, dtype = tf.float32)
Amp = tf.constant(1, dtype = tf.float32)
frequency = tf.constant(1, dtype = tf.float32)
sigma = tf.constant(0.5, dtype = tf.float32)
source_x_coord = tf.constant(0, dtype = tf.float32)
Gaussian_impulse = Amp * tf.exp(-(1/(sigma**2))*(x-source_x_coord)**2)
S = Gaussian_impulse * tf.sin( 1 * tf.constant(math.pi) * frequency * t )
u = u_model(tf.concat([x,t], 1))
u_x = tf.gradients(u,x)
u_xx = tf.gradients(u_x, x)
u_t = tf.gradients(u,t)
u_tt = tf.gradients(u_t,t)
f_u = u_xx - (1/c**2) * u_tt + S
return f_u
The results shown are good but we can reach a lower L2 error value.
@engsbk I would ask the same question here - what's your training horizon? The 1D Wave w/source actually look pretty good to me! Of course there's room for improvement but that is impressive for a first pass at it.
The 2D case is tougher - that one may require some creativity. Try adding in self-adaptive weighting and see if that coaxes your residual errors to decrease a little better. There may also be an issue in the f_model definition but it looks reasonable to me, so that's just a guess. Weighting the IC a little heavier initially is usually a good starting point with SA weights.
Layer (type) Output Shape Param #
=================================================================
dense (Dense) (None, 64) 256
dense_1 (Dense) (None, 64) 4160
dense_2 (Dense) (None, 64) 4160
dense_3 (Dense) (None, 64) 4160
dense_4 (Dense) (None, 1) 65
=================================================================
Total params: 12,801
...
Non-trainable params: 0
_________________________________________________________________
None
Starting Adam training
Adam epoch 20000: 100%|██████████| 20000/20000 [07:12<00:00, 46.28it/s, loss=0.000213]
Starting L-BFGS training
Executing eager-mode L-BFGS
L-BFGS epoch 20000: 100%|█████████▉| 19999/20000 [28:47<00:00, 11.58it/s, loss=0.000112]
For the 2D problem, the loss had a low value, but the comparison results were not accurate. I'm not sure what do you mean by training horizon...
Sure this can result from it finding the 0 solution, weighting the IC with SA weights should help.
By training horizon I just mean the number of training iterations of Adam/BFGS. 20k is fine, in this case there's likely another issue with the 2D case. I bet if you dial down the adam learning rate and increase the number of training iterations on the 1D case you'll improve it.
The 2D case is tougher - that one may require some creativity. Try adding in self-adaptive weighting and see if that coaxes your residual errors to decrease a little better. There may also be an issue in the f_model definition but it looks reasonable to me, so that's just a guess. Weighting the IC a little heavier initially is usually a good starting point with SA weights.
Good idea, I'll try changing the learning rate and IC weight and keep you posted with the results. Is there a tdq command for modifying the learning rate and weights instead of editing the env files?
I just want to make sure Am I changing the IC weight using the correct lines:
def func_ic(x,y):
return tdq.utils.constant(0)
init = IC(Domain, [func_ic], var=[['x','y']])
upper_x = dirichletBC(Domain, val=0.0, var='x', target="upper")
lower_x = dirichletBC(Domain, val=0.0, var='x', target="lower")
upper_y = dirichletBC(Domain, val=0.0, var='y', target="upper")
lower_y = dirichletBC(Domain, val=0.0, var='y', target="lower")
BCs = [init, upper_x, lower_x, upper_y, lower_y]
def f_model(u_model, x, y, t):
c = tdq.utils.constant(1)
Amp = tdq.utils.constant(2)
freq = tdq.utils.constant(1)
sigma = tdq.utils.constant(0.2)
source_x = tdq.utils.constant(0.5)
source_y = tdq.utils.constant(2.5)
GP = Amp * tf.exp(-0.5*( ((x-source_x)/sigma)**2 + ((y-source_y)/sigma)**2 ))
S = GP * tf.sin( 2 * tdq.utils.constant(math.pi) * freq * t )
u = u_model(tf.concat([x,y,t], 1))
u_x = tf.gradients(u,x)
u_xx = tf.gradients(u_x, x)
u_y = tf.gradients(u,y)
u_yy = tf.gradients(u_y, y)
u_t = tf.gradients(u,t)
u_tt = tf.gradients(u_t,t)
f_u = u_xx + u_yy - (1/c**2) * u_tt + S
return f_u
## Which loss functions will have adaptive weights
# "residual" should a tuple for the case of multiple residual equation
# BCs have to follow the same order as the previously defined BCs list
dict_adaptive = {"residual": [True],
"BCs": [True, False, False, False, False]}
## Weights initialization
# dictionary with keys "residual" and "BCs". Values must be a tuple with dimension
# equal to the number of residuals and boundary conditions, respectively
init_weights = {"residual": [tf.random.uniform([N_f, 1])],
"BCs": [10 * tf.random.uniform([100,1,1,1,1]) ,None]}
Also, I'm not sure how to change the learning rate, if you can help with this too, it'll be great.
I've increased the collocation points just in case they're not enough.
N_f = 60000
Please advise.
This looks correct to me. Modification of the learning rate can be done by overwriting the default optimizer with another Adam optimizer with a different lr. See here for how to do so - https://tensordiffeq.io/hacks/optimizers/index.html
This looks correct to me. Modification of the learning rate can be done by overwriting the default optimizer with another Adam optimizer with a different lr. See here for how to do so - https://tensordiffeq.io/hacks/optimizers/index.html
Thanks! Before I read this page I just added tf_lr=0.005
and newton_lr=0.8
to fit()
, fit_dist()
, and compile()
.
I think what you referred to is a better generalization. Just In case other optimizers are to be tested.
Changing tf_optimizer
, does that change the first optimizer and the second is still BFGS?
Edit: Nevermind, I realized I can control that with newton_iter
.
Thank you for the informative documentation!
I was trying to follow the example of self-adaptive training in https://tensordiffeq.io/model/sa-compiling-example/index.html, but I got this error:
TypeError Traceback (most recent call last)
c:\Users\User\OneDrive - The Pennsylvania State University\Research-MacBook Pro\Deep Learning\TensorDiffEq-main\examples\Wave2D_new.ipynb Cell 10' in <cell line: 19>()
16 layer_sizes = [3, 32, 32, 32, 32, 32, 32, 1]
18 model = CollocationSolverND()
---> 19 model.compile(layer_sizes, f_model, Domain, BCs, isAdaptive=True,
20 col_weights=col_weights, u_weights=u_weights)
TypeError: compile() got an unexpected keyword argument 'col_weights'
I'm not sure if I'm using an older version of compile()
. When I checked models.py
, it seems like it's expecting dict_adaptive
and init_weights
expecting instead of col_weights
and u_weights
.
Any suggestions on how to fix this?
I was trying to follow the example of self-adaptive training in https://tensordiffeq.io/model/sa-compiling-example/index.html, but I got this error:
TypeError Traceback (most recent call last) c:\Users\User\OneDrive - The Pennsylvania State University\Research-MacBook Pro\Deep Learning\TensorDiffEq-main\examples\Wave2D_new.ipynb Cell 10' in <cell line: 19>() 16 layer_sizes = [3, 32, 32, 32, 32, 32, 32, 1] 18 model = CollocationSolverND() ---> 19 model.compile(layer_sizes, f_model, Domain, BCs, isAdaptive=True, 20 col_weights=col_weights, u_weights=u_weights) TypeError: compile() got an unexpected keyword argument 'col_weights'
I'm not sure if I'm using an older version of
compile()
. When I checkedmodels.py
, it seems like it's expectingdict_adaptive
andinit_weights
expecting instead ofcol_weights
andu_weights
.Any suggestions on how to fix this?
This is old syntax - sorry for the confusion. Your original script was correct, i.e. defining dict_adaptive. That was a recent change in syntax that needs updating still in some places in the documentation
Changing tf_optimizer, does that change the first optimizer and the second is still BFGS?
Edit: Nevermind, I realized I can control that with newton_iter.
The only supported second order solver is L-BFGS, newton_iter
just controls the number of training epochs
Hello @levimcclenny ,
Thanks for your suggestions. I tried increasing Adam epochs to 100000, but the results are still not good enough. Please have a look at my setup and tell me your feedback, please.
import math
import scipy.io
import tensordiffeq as tdq
from tensordiffeq.boundaries import *
from tensordiffeq.models import CollocationSolverND
!nvidia-smi
Domain = DomainND(["x", "y", "t"], time_var='t')
Domain.add("x", [0.0, 5.0], 100)
Domain.add("y", [0.0, 5.0], 100)
Domain.add("t", [0.0, 5.0], 100)
N_f = 100000
Domain.generate_collocation_points(N_f)
def func_ic(x,y):
return 0
init = IC(Domain, [func_ic], var=[['x','y']])
upper_x = dirichletBC(Domain, val=0.0, var='x', target="upper")
lower_x = dirichletBC(Domain, val=0.0, var='x', target="lower")
upper_y = dirichletBC(Domain, val=0.0, var='y', target="upper")
lower_y = dirichletBC(Domain, val=0.0, var='y', target="lower")
BCs = [init, upper_x, lower_x, upper_y, lower_y]
def f_model(u_model, x, y, t):
c = tdq.utils.constant(1)
Amp = tdq.utils.constant(2)
freq = tdq.utils.constant(1)
sigma = tdq.utils.constant(0.2)
source_x = tdq.utils.constant(0.5)
source_y = tdq.utils.constant(2.5)
GP = Amp * tf.exp(-0.5*( ((x-source_x)/sigma)**2 + ((y-source_y)/sigma)**2 ))
S = GP * tf.sin( 2 * tdq.utils.constant(math.pi) * freq * t )
u = u_model(tf.concat([x,y,t], 1))
u_x = tf.gradients(u,x)
u_xx = tf.gradients(u_x, x)
u_y = tf.gradients(u,y)
u_yy = tf.gradients(u_y, y)
u_t = tf.gradients(u,t)
u_tt = tf.gradients(u_t,t)
f_u = u_xx + u_yy - (tdq.utils.constant(1)/c**2) * u_tt + S
return f_u
## Which loss functions will have adaptive weights
# "residual" should a tuple for the case of multiple residual equation
# BCs have to follow the same order as the previously defined BCs list
dict_adaptive = {"residual": [True],
"BCs": [True, False, False, False, False]}
## Weights initialization
# dictionary with keys "residual" and "BCs". Values must be a tuple with dimension
# equal to the number of residuals and boundary conditions, respectively
init_weights = {"residual": [tf.random.uniform([N_f, 1])],
"BCs": [100 * tf.random.uniform([100,1,1,1,1]) ,None]}
layer_sizes = [3, 40,40,40,40,40,40,40, 1]
model = CollocationSolverND()
model.compile(layer_sizes, f_model, Domain, BCs)#, isAdaptive=True,
#dict_adaptive=dict_adaptive, init_weights=init_weights)
model.tf_optimizer = tf.keras.optimizers.Adam(learning_rate = 5e-3)
model.tf_optimizer_weights = tf.keras.optimizers.Adam(learning_rate = 5e-3)
model.fit(tf_iter=100000, newton_iter=10000)
#######################################################
#################### PLOTTING #########################
#######################################################
data = np.load('wave2D_1Element.npz')
Exact = data['u_output']
Exact_u = np.real(Exact)
x = Domain.domaindict[0]['xlinspace']
y = Domain.domaindict[1]['ylinspace']
t = Domain.domaindict[2]["tlinspace"]
X, Y, T = np.meshgrid(x, y, t)
X_star = np.hstack((X.flatten()[:, None], Y.flatten()[:, None], T.flatten()[:, None]))
u_star = Exact_u.T.flatten()[:, None]
u_pred, f_u_pred = model.predict(X_star)
error_u = tdq.helpers.find_L2_error(u_pred, u_star)
print('Error u: %e' % (error_u))
lb = np.array([0.0, 0.0, 0.0])
ub = np.array([5.0, 5.0, 5])
tdq.plotting.plot_solution_domain2D(model, [x, y, t], ub=ub, lb=lb, Exact_u=Exact_u)
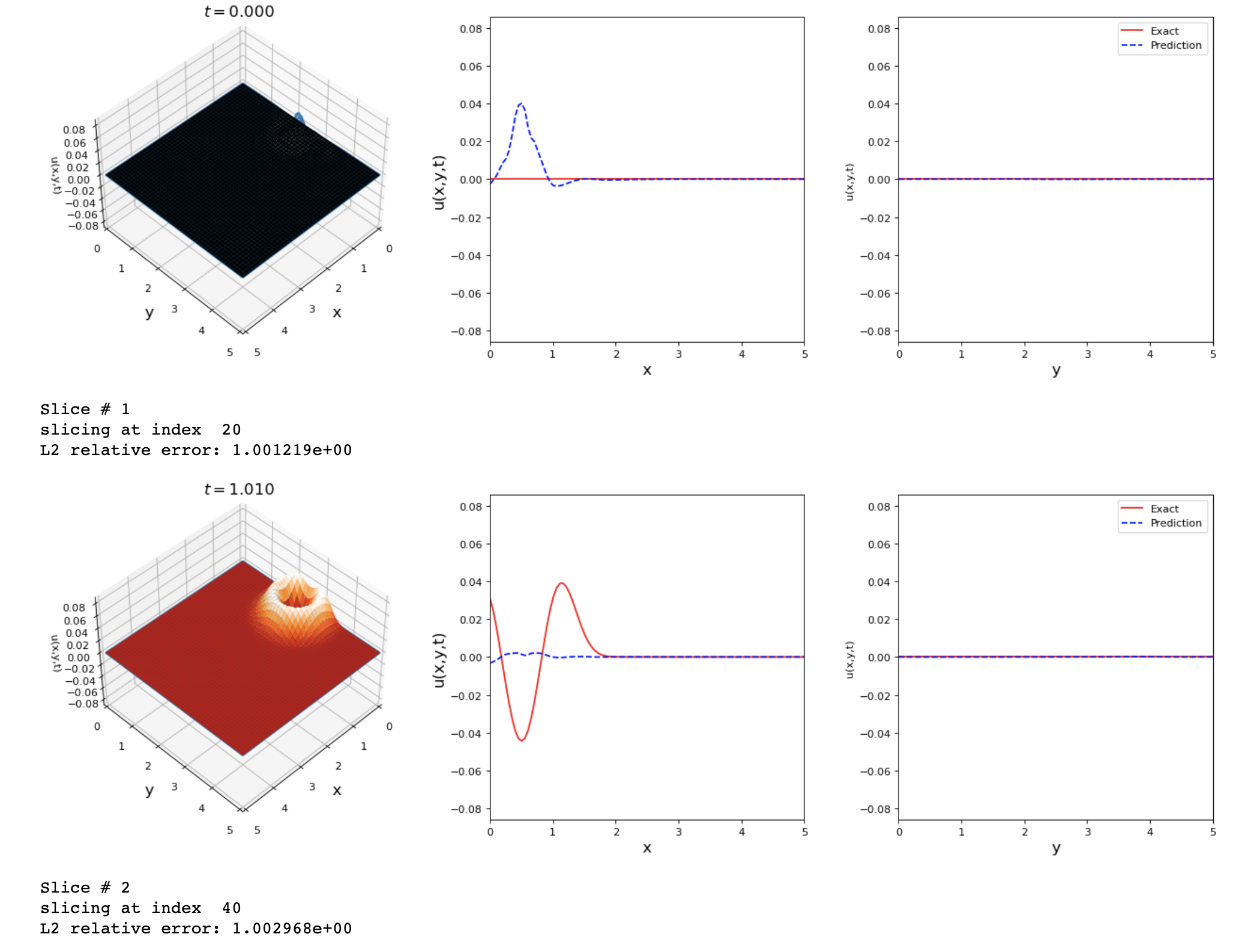
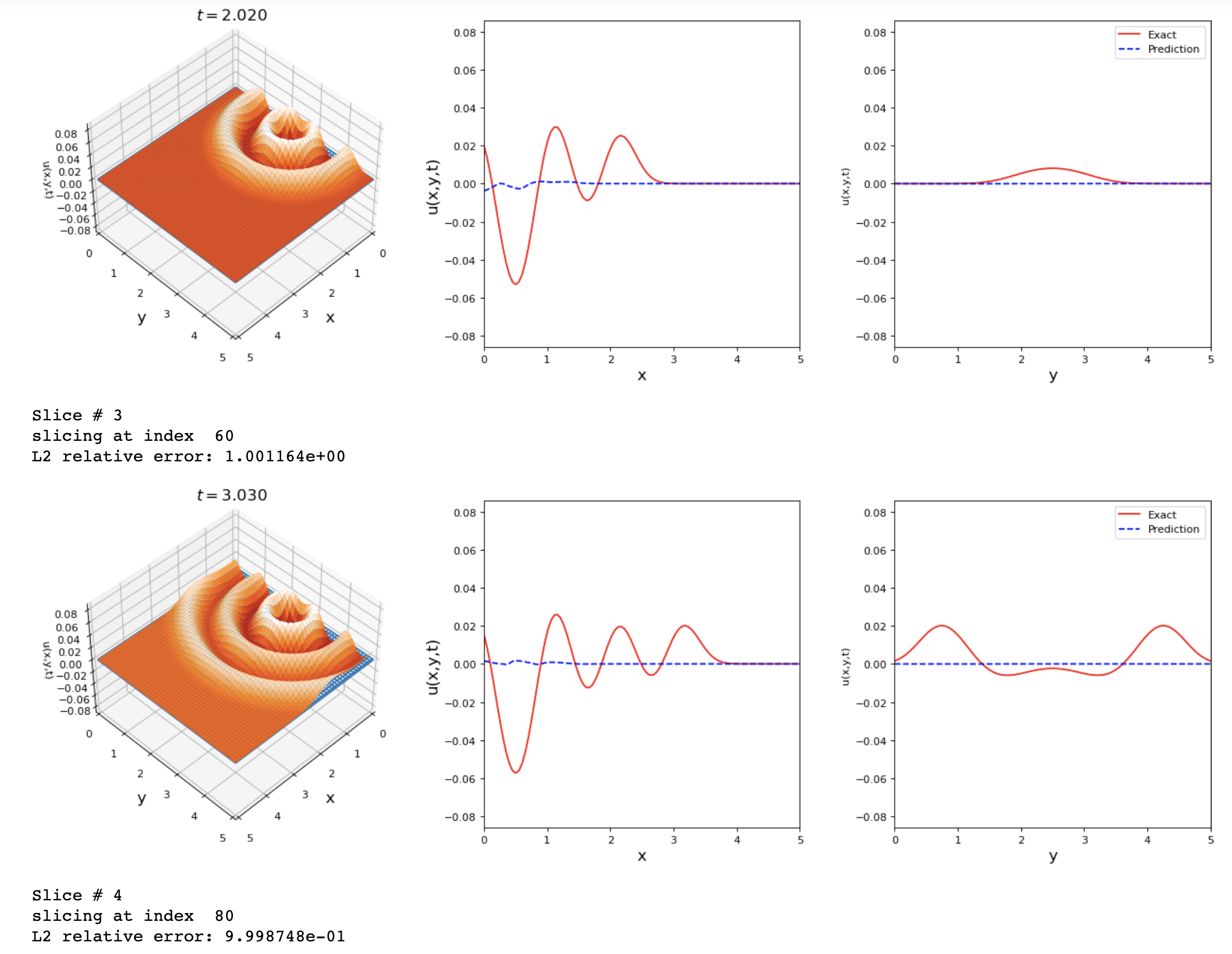
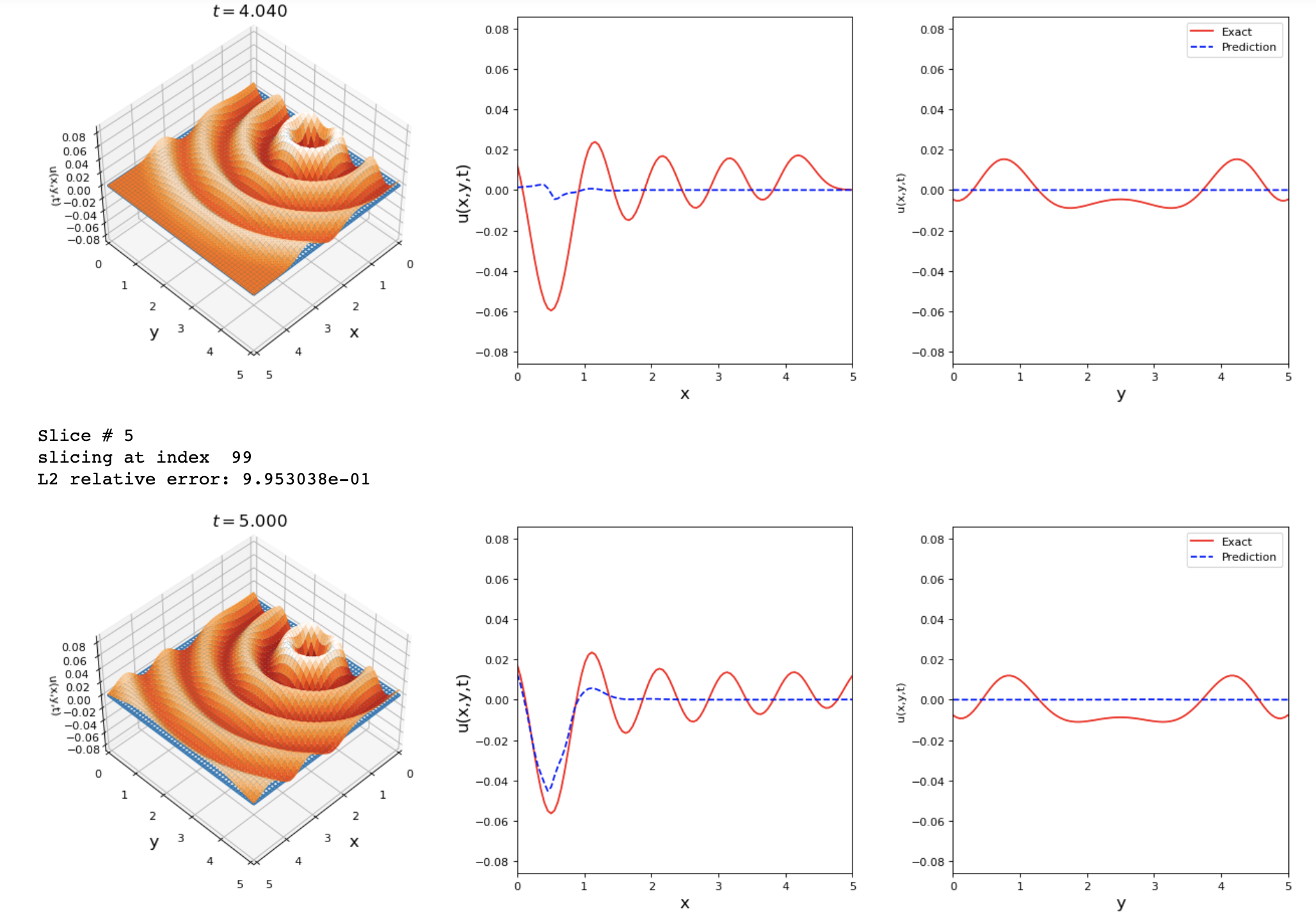
I avoided using SA because the loss kept increasing until nan
if I implement it in the model.
Looking forward to your reply. Thanks!