evan
evan copied to clipboard
:mag: python package to evaluate GANs for video generation
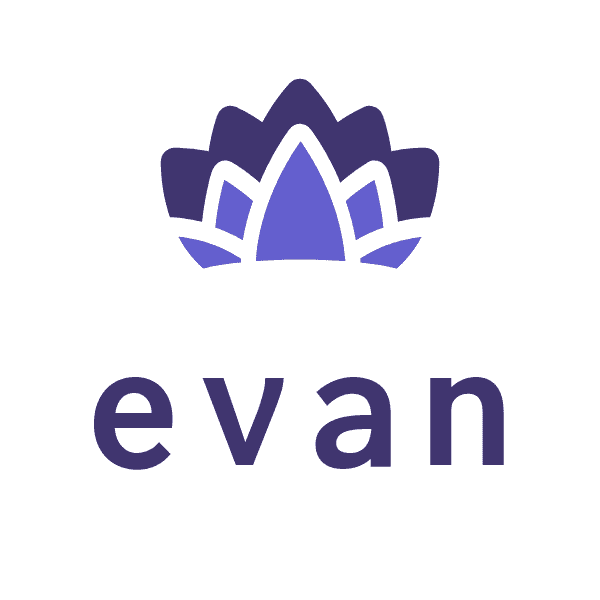
Library and CLI tool for evaluating video GANs
"Evan" automates GAN evaluation for video generation. The library gives two advantages for you.
- You don't need to prepare the Inception Model and process your samples for evaluation.
- You don't need to find source codes or write algorithm of each GAN metric.
Now, evan supports following metrics.
- Inception Score [1]
- Frechet Inception Distace [2]
- Precision and Recall for Distributions [3]
Installation
Required
- Python 3.6 or higher
- FFmpeg
$ pip install evan
Example
See examples directory or docs for details.
import torch
from pathlib import Path
import skvideo.io
import evan
gen = YourGenerater()
N = 5000
batchsize = 100
temp = tempfile.TemporaryDirectory()
temp_dir = Path(temp.name)
fps = 30
# generate video samples by your GAN and
# save them into a directory in .mp4
for batch in range(0, N, batchsize):
xs = gen.generate(batchsize) # (B, T, H, W, C)
for i, x in enumerate(xs):
path = str(temp_dir / f"{i}.mp4")
writer = skvideo.io.FFmpegWriter(path, inputdict={"-r": str(fps)})
for frame in video:
writer.writeFrame(frame)
# compute Inception Score by using evan
score = evan.compute_inception_score(
temp_dir, batchsize=batchsize, verbose=True
)
# >> found 10000 samples.
# >> converting videos into conv features using inception model (on gpu)...
# 100%|█████████████████████████████████| 10000/10000 [XX:XX<XX:XX, XX.XX s/it]
# >> computing IS...
# generated samples: '/var/folders/k4/xxx/probs.npy'
print(score)
temp.cleanup()
CLI
❯ evan -h
usage: evan [-h] {compute,plot} ...
a tool for evaluation video GANs.
optional arguments:
-h, --help show this help message and exit
subcommands:
command names.
{compute,plot}
compute compute evaluation score.
plot visualize evaluation result.
Details
Evaluation flow
WIP
Inception Model
WIP
Reference
- [1] "Improved Techniques for Training GANs", https://arxiv.org/abs/1606.03498
- [2] "GANs Trained by a Two Time-Scale Update Rule Converge to a Local Nash Equilibrium", https://arxiv.org/abs/1706.08500
- [3] "Assessing Generative Models via Precision and Recall", https://arxiv.org/abs/1806.00035
- [4] "An empirical study on evaluation metrics of generative adversarial networks", https://arxiv.org/abs/1806.07755