SincNet
SincNet copied to clipboard
SincNet is a neural architecture for efficiently processing raw audio samples.
I'm using your model to train on TIMIT, my parameters are the same as yours ,such as lr、batchsize. I use the script named ‘speaker_id.py ’ to train model, and ‘compute_d_vector.py’...
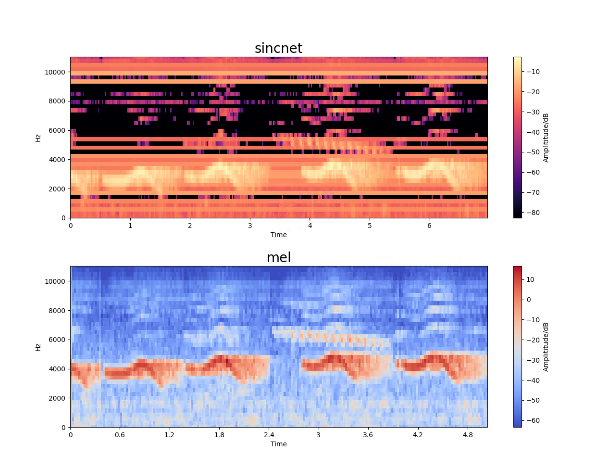
Hi Mirco, can you please upload cfg file or data preprocessing method for Voxceleb dataset? Using cfg file of Librispeech or Timit yields a very high EER.
Hi and thank you for sharing the code! I was studying the creation of the Sinc filterbank in the SincConv_fast class and I have a question about this section: ```...
Hello Mirco Ravanelli, Training SincNet for speaker-id using TIMIT data following your directions, with the config file provided in your github end up with a different cumulative frequency response. The...
numpy.save has the allow_pickle=True as default while numpy.load has allow_pickle=False, so this raises an error that can be fixed by explicitly setting the allow_pickle variable.
The current LibriSpeech configuration throws an error due to the missing third variable for fc_drop. We can either reduce the number of the fully connected layer (fc_lay) or increase the...
I had the problem of running the code and got nan from the loss function after 2-3 iterations. While testing the problem I saw that some parameters such as gamma...
I test the performance of your pretrained model on the famous dataset VoxCeleb1(http://www.robots.ox.ac.uk/~vgg/data/voxceleb/). The EER I got is 30%. I used the compute_d_vector.py to get the d_vector of the audio...
https://github.com/mravanelli/SincNet/blob/master/dnn_models.py#L106-L108 : ```python #self.window_ = torch.hamming_window(self.kernel_size) n_lin=torch.linspace(0, (self.kernel_size/2)-1, steps=int((self.kernel_size/2))) # computing only half of the window self.window_=0.54-0.46*torch.cos(2*math.pi*n_lin/self.kernel_size); ``` Could it be replaced instead by `self.window_ = torch.hamming_window(kernel_size)[:kernel_size // 2]`? Or...