WeeklyArxivTalk
WeeklyArxivTalk copied to clipboard
[20230108] Weekly AI ArXiv 만담 시즌2 - 1회차
News
- Conferences
- AAAI 2023 모두들 축하드립니다 (뒷북)
- ACL 2023: Softconf 1월 17일
- ICML 2023: Openreview 1월 26일 (한국 시간 27일 새벽 5시.. )
- CVPR 2023: 리뷰 1월 10일까지.. Aㅏ.....
- ICML 2023에서 ChatGPT 사용과 관련한 이슈
- Anthropic 의 Claude 가 ChatGPT3 보다 낫다는데...
- https://twitter.com/goodside/status/1611456645737107456?s=20&t=mT035MdDp9zDpowONheydg
-
Constitutional AI: Harmlessness from AI Feedback
- CES 2023 대해부 웨비나
Research
-
Neural Codec Language Models are Zero-Shot Text to Speech Synthesizers
- MS에서 공개된 DALL-E 스타일의 Zero-shot Text-to-speech version. (음성 스타일 + 화자)
- Of-the-shelf audio encoder --> VQ token --> Neural Codec LM --> Conditional LM token 생성 --> Audio decoder
- Text prompt --> Phoneme conversion token, Audio prompt --> audio codec encoder token
- 프로젝트 & 데모: https://valle-demo.github.io/
-
Muse: Text-To-Image Generation via Masked Generative Transformers
- Google에서 나온 Transformer 기반 Masked Image Model 스타일 Text-to-image generation
- VQ token 레벨의 Masking. Text encoder (T5-XXL), Base MIM Transformer, HR Superresolution Transformer,
- 기존 Pixel level diffusion (Imagen, DALLE2) 나 Parti (autoregressive) 보다 이래 저래 효율적이라는..
- 물론 VQ tokenizer는 필수. Masking ratio는 Cosine scheduling (MaskGIT)
-
흥미있는 연구
-
Large Language Models as Corporate Lobbyists
- 제목 그대로.. 대기업의 B2B SaaS 상품화??
- Scalable Diffusion Models with Transformers: LDM의 Attention UNet 을 Transformer로
- Cool Japan Animation by FT Stable Diffusion: 당신이 애니덕후라면..
-
Large Language Models as Corporate Lobbyists
Research (겸 뉴스?): Large Language Models Encode Clinical Knowledge
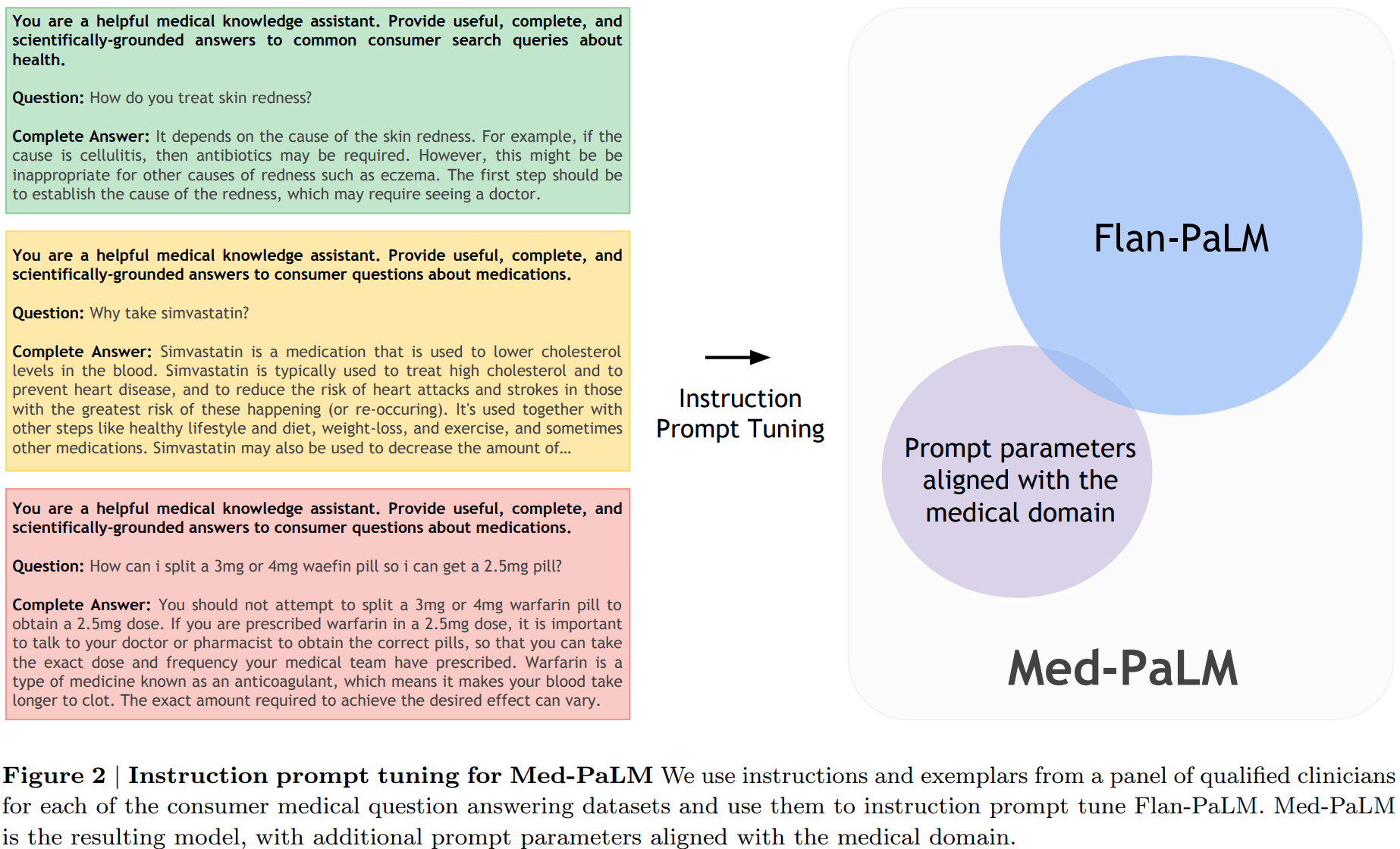
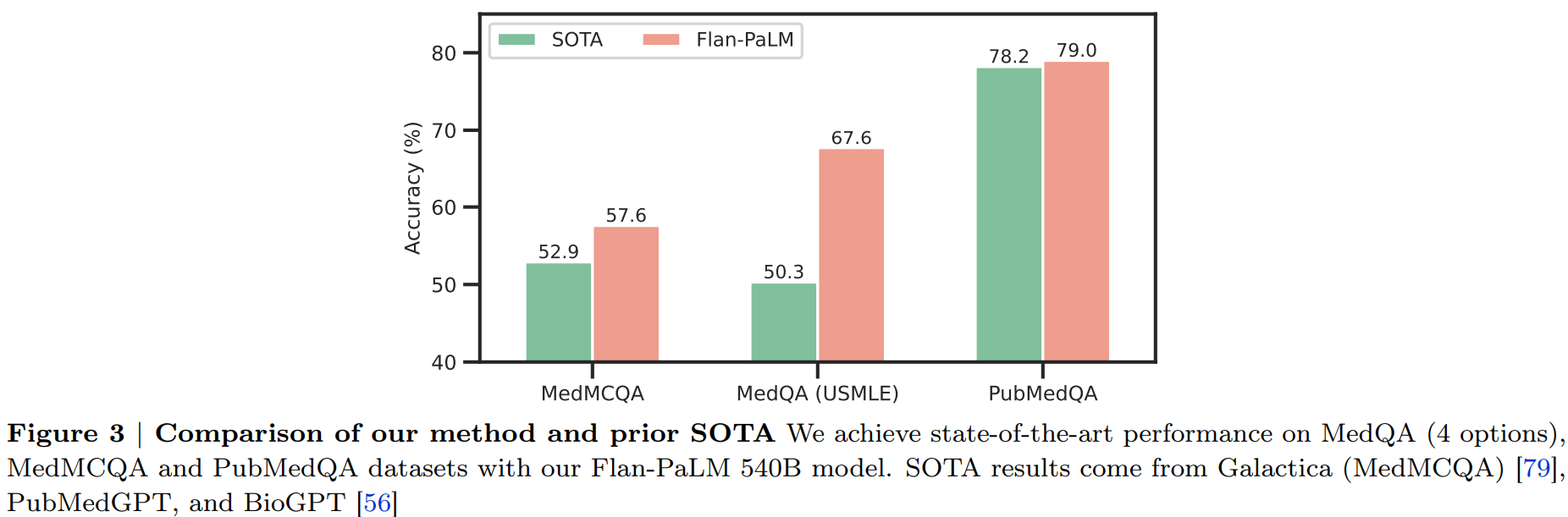
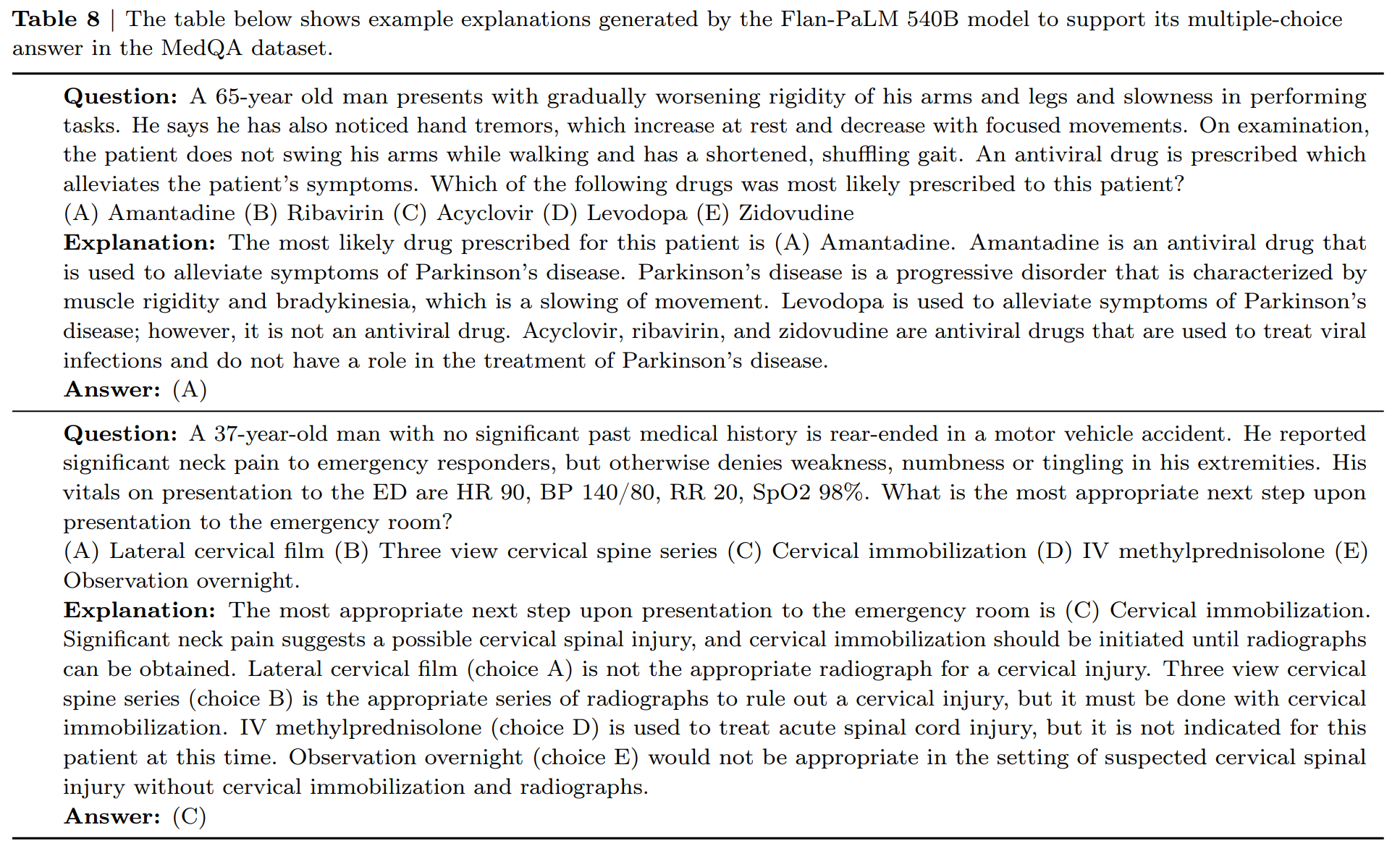
구글과 딥마인드에서 ChatGPT와 유사하게 의료 영역에서 질의응답에 답변을 할 수 있도록 초거대 언어 모델을 학습한 논문입니다. ChatGPT의 등장으로 LLM을 여러 영역에서 사용하는데 관심이 급증하면서 연구가 활발해질 것으로 보입니다.
ChatGPT와는 다르게 RLHF(Reinforcement Learning with Human Feedback)을 사용하지 않고 Instruction fine-tuning 기반의 FLAN 모델에서 soft prompt, 단어 대신 한 단계 encoding된 prompt를 학습한 후 추가하는 방식을 적용합니다.
GitHub: https://github.com/mjbommar/gpt-takes-the-bar-exam
유사한 맥락으로 법률 분야에서 GPT 3.5 계통의 모델을 미국 변호사 시험 모의고사에서 객관식 문항에 적용했을 때 별도의 학습 없이 prompt tuning 만으로도 상당한 성능을 얻을 수 있는 것을 보여줍니다.
두 논문은 LLM을 전문 지식이 필요한 분야에서 적용할 때 신규 학습 뿐만 아니라 prompting 방법을 적용하는 것의 중요성과 전문성을 유도하는 방법에 대한 실험적 연구로 의미가 있다고 생각됩니다.
Tutorial High-Performance Computing for Deep Learning (HPC4DL)
딥러닝, 특히 PyTorch 사용자를 위한 GPU 하드웨어부터 최상위 소프트웨어 기술스택까지 어떻게 구성되어있는지 발표를 한 영상을 공유해드립니다. 딥러닝 학습의 원리 및 GPU 하드웨어에서의 구현, PyTorch에서 적용되는 방법 등 깊이 있게 다룰 뿐만 아니라 실제 연구개발을 하시는 분들께 도움이 될만한 팁과 경험 또한 많이 공유했습니다.
Research
-
ResGrad: Residual Denoising Diffusion Probabilistic Models for Text to Speech
- Keyword : diffusion, denoising, TTS, enhancement
- Sample URL : https://resgrad1.github.io/
-
Motivation / Goal / Contribution
- Motivation 기존의 diffusion을 이용한 TTS는 고품질의 sample을 만들어낼 수 있지만, 합성 속도가 느리기 때문에 실제 real-time application에 사용하기 어려웠고, 경량화 모델은 스텝 수를 줄여서 경량화했지만 대신 품질이 떨어짐.
- Goal
이 논문에서는 품질을 유지한 채, 미리 학습한 TTS의 mel-spectrogram을 가벼운 diffusion 모델로 학습하여 품질을 올리는 방법을 사용하여 합성 속도가 빠르면서도 품질이 우수한 모델을 개발하는 것을 목표. - Contribution
전체 speech가 아닌 residual 값만 예측하기 위해 DDPM 모델을 사용함으로써 효율적으로 output 성능을 높이면서도 빠른 합성 속도를 가지는 모델을 만들 수 있음.
-
Proposed Method
- 미리 학습한 TTS에서 합성한 mel-spectrogram과 gt mel-spectrogram 사이의 residual 값을 ResGrad 모듈로 학습.
- TTS로 합성한 smoothing된 mel-spectrogram을 refine하여 gt에 가깝게 만드는 방법을 제안.
- DDPMs을 위한 학습 방법은 Grad-TTS를 따라 만듦.
-
Experiment / Results
- parallel하게 만들어내는 FastSpeech2의 경우 굉장히 빠른 합성 속도를 가지지만 3.29 수준으로 MOS가 낮음
- single 화자의 경우 GradTTS-50와 ResGrad-50간의 MOS 차이는 없지만 multi-speaker의 경우 ResGrad의 성능이 좋음
-
Modeling the Rhythm from Lyrics for Melody Generation of Pop Song
- ISMIR 2022 / ByteDance
- Keyword : Melody generation, Lyric to melody
-
Motivation / Goal / Contribution
- Motivation end-to-end lyric-to-melody model은 많은 양의 paired dataset이 필요하여 만들기 어려움
- Goal lyric-to-melody model을 두 개의 step으로 나누어 lyric-to-rhythm, rhythm-to-melody으로 two-stage로 중국어에 대한 lyric-to-melody generation을 수행
- Contribution lyric-to-rhythm, rhythm-to-melody로 나누는 새로운 접근법을 제안하였고 다른 시스템과 비교하여 긴 text에 대해서 좋은 성능을 보였음
-
Proposed Method
- (Figure 1) Lyric-to-rhythm module을 통하여 rhythm을 만들고 chord 진행을 입력받아서 melody를 생성
- (Figure 3, 4) lyric-to-rhythm module은 가사가 입력으로 들어오면 POS tagging을 하고 가사와 tag를 transformer encoder, decoder를 이용하여 rhythm feature(one-hot vector)을 생성 (autoregressive 방법)
- (Figure 2) Chord-conditioned Melody Transformer (CMT) 에서 chord 진행으로 rhythm을 생성하고 chord와 rhythm으로 pitch를 만들어 최종 melody를 생성
-
Experiment / Results
- 데이터 셋
- lyric-to-rhythm model : 45K Chinese pop songs을 온라인에서 lyric과 audio pair를 크롤링
- rhythm-to-melody model : 30K 마디의 음악, POP909, Lead-Sheet-Dataset을 사용
- 평가
- Rhythm, Harmony, Melody, Overall로 나누어 1~5점으로 평가
- 평가가 TeleMelody를 능가하거나 비슷한 수준
- 두 모델 모두 'melody가 이상하다', '너무 반복이 많다' 라는 평가가 있었지만, TeleMeoldy만 '리듬이 이상하다'는 평을 받았음
- 데이터 셋
흥미로운 연구
-
Language Models are Drummers: Drum Composition with Natural Language Pre-Training
- automatic music generation은 많은 양의 데이터가 필요하지만 얻기는 어려움
- 이 논문에서는 GPT3를 드럼에 대한 수천개의 미디 파일로 finetuning하여 reasonable한 drum groove를 만듦
- 평가하는 부분도 제안을 하고 있고 실제 전문가들이 만든 것과 비교를 하는 것으로 보임 (자세한 것은 논문 참조)
- Groove MIDI Dataset
논문
Scaling Language-Image Pre-training via Masking
- META AI, FAIR
- CLIP과 MAE의 만남
- image encoding 시에 50%(혹은 75%) masking 하고, unmasked patch 만으로 encoding 후 CLIP을 적용
- 아주아주 단순한 아이디어이지만 gain은 꽤 괜찮음
- 실험을 많이 하긴 했지만, 다른 task에 다 적용 가능할지?
TinyMIM: An Empirical Study of Distilling MIM Pre-trained Models
- MSRA
- MIM(Masked Image Modeling)이 대중적으로 많이 사용되지만, 작은 model에는 잘 동작하지 않는 문제를 지적
- 다른 approach들은 inductive bias를 넣는 방식으로 해결하려 했으나, 이 논문에서는 distillation을 활용하여 MIM의 이득을 작은 model에서도 가져갈 수 있는 방법을 고안
- 다양한 실험을 통해서 distillation을 위한 bag of tricks를 정리
- distillation targets: token relation을 distillation 하는게 CLS token이나 feature map을 하는 것보다 좋음
- teacher와 다른 downstream task일 경우 last layer보다 중간 layer가 더 좋음
- original image가 masked image보다 좋고, regulrization은 아주 살짝만
- auxiliary losses는 도움 안됨
- ViT-B -> ViT-T 로 한방에 가는 것보다 ViT-B -> ViT-S -> ViT-T로 순차적으로 가는게 더 잘됨
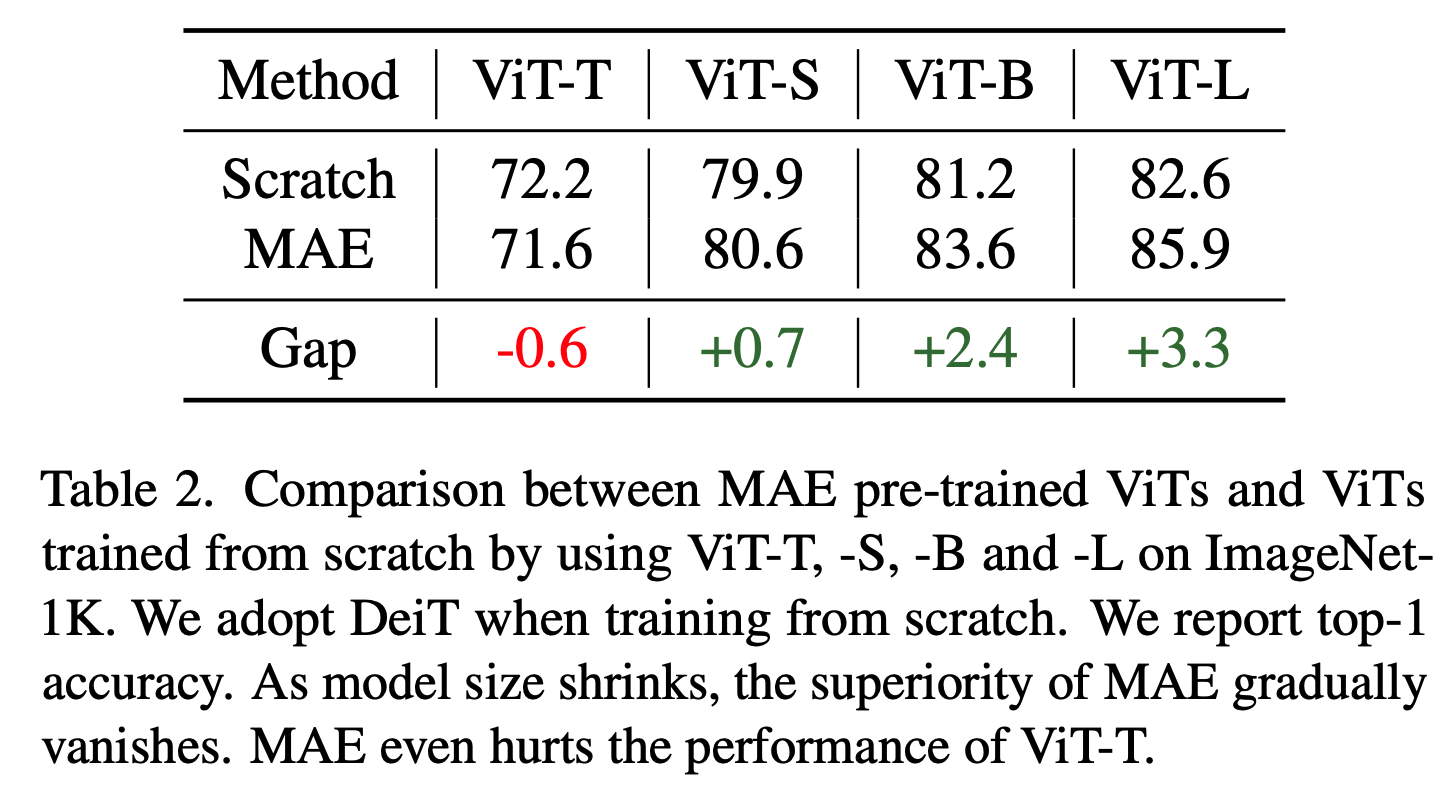
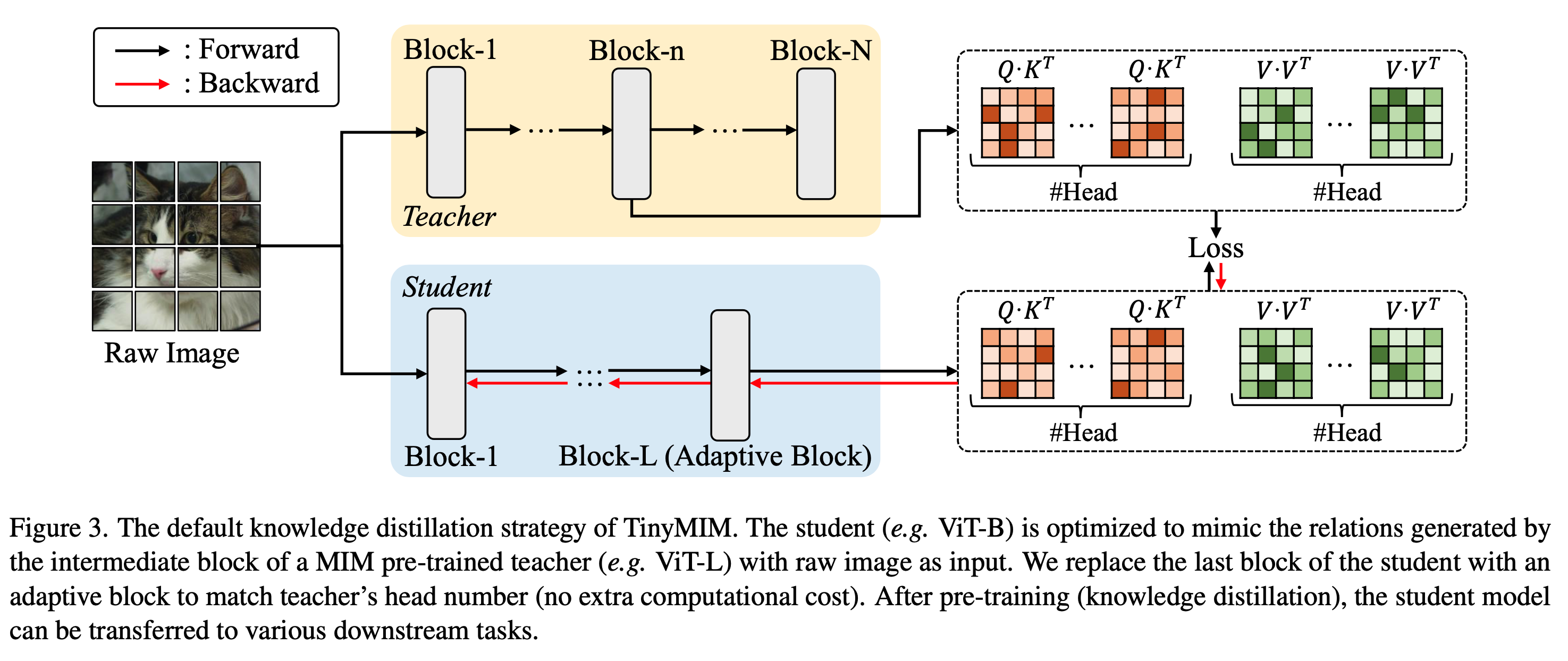
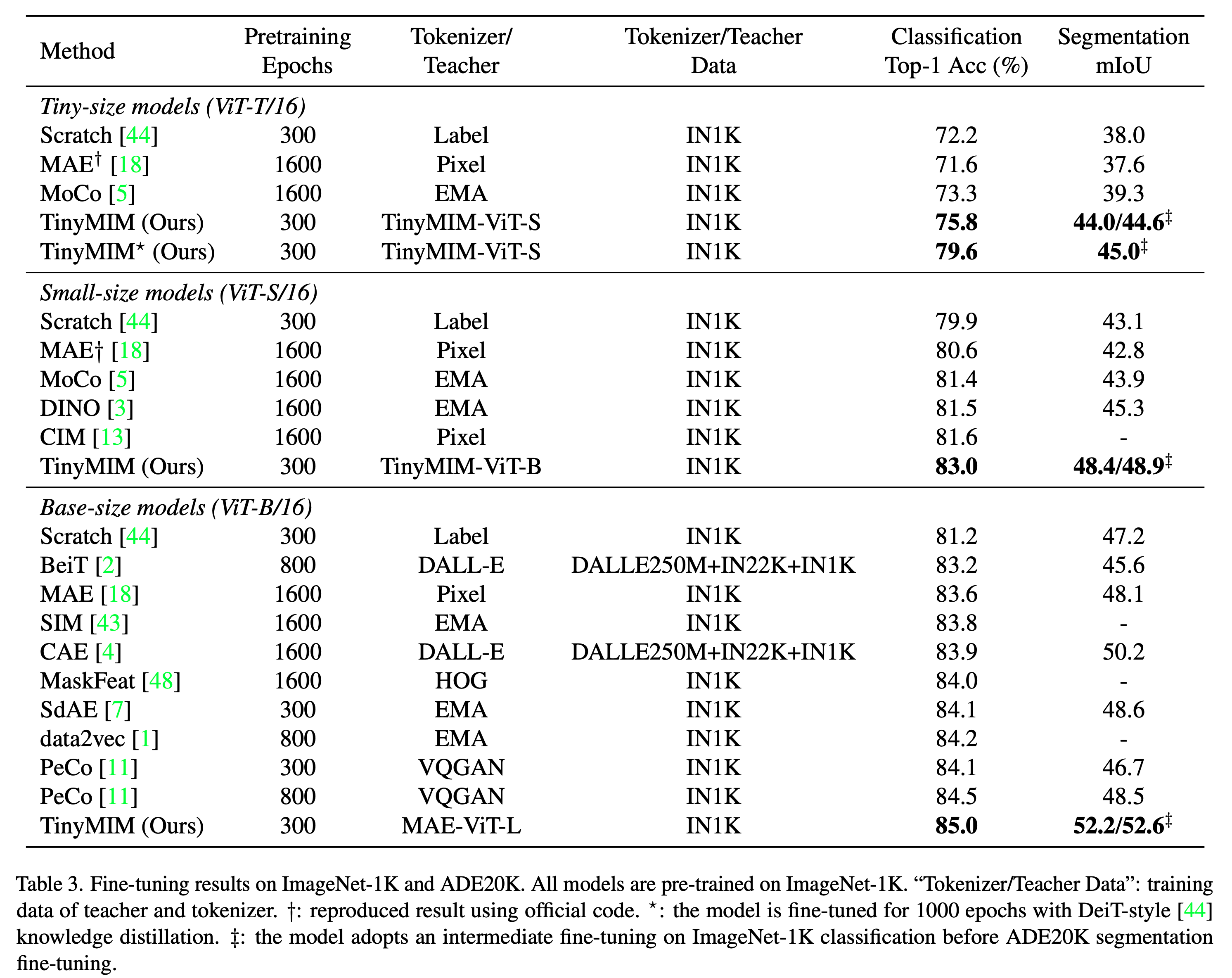
ConvNeXt V2: Co-designing and Scaling ConvNets with Masked Autoencoders
- 우상현님(KAIST) 1저자, FAIR, New York University
- ConvNext에 MAE를 적용하기 위한 방법
- Sparse convolution + Global Response Normalization
News (AI)
- ALT 2023 Accepted Papers: http://algorithmiclearningtheory.org/alt2023/accepted-papers/
News (Math)
-
A non-constructive proof of the Four Colour Theorem (Jackson & Richmond, arXiv 2022)
- 현재 증명: computer-assisted proof (Appel & Haken, 1989)
- error found! https://mathstodon.xyz/@noamzoam/109567981846531700 -RL과 엮을 수 있는 건덕지...? (cf. Constructions in combinatorics via neural networks)
AI Papers
-
Cramming: Training a Language Model on a Single GPU in One Day (Geiping & Goldstein, arXiv 2022) - under review for ICLR 2023
- Tom Goldstein옹의 논문 (Univ of Maryland)
- TLDR: GPU 하나 (RTX2080 Ti or A4000)를 이용해서 하루만에 얼마나 할 수 있을까요
- 엄청난 trick들의 항연! twitter summary
-
Benchmarking Graph Neural Networks (Dwivedi et al., JMLR 2023)
- open source GNN benchmarking framework!
- 12개의 dataset들 (real-world, synthetic), PyTorch+DGL을 기반, parameter budgets for a fair comparison,
- https://github.com/graphdeeplearning/benchmarking-gnns
- open source GNN benchmarking framework!
-
Named Tensor Notation (Chiang et al., TMLR 2023)
- Twitter link
- https://namedtensor.github.io/
- AI notation을 정리하려는 시도
- 어느 axis로 operation을 취하는지 명확히 하기, diff calculus 및 lin alg 확실히 정리... 등등
-
The Forward-Forward Algorithm (Geoffrey Hinton, arXiv 2022)
- backward prop 없이 배우는 법!
- NeurIPS 2022에서 Hinton옹이 발표함
- Layerwise tuning of parameters for positive/negative samples
-
Editing Models with Task Arithmetic (Ilharco et al., arXiv 2022) - under review for ICLR 2023
- easy control of pre-trained models! (bias reduction, alignment with humans, improved accuracies...)
- direct editing in weight space!
- ex. fine-tuning GPT-2 on hate speech, then negating the resulting task vector results in 6x less toxic generation
Non-AI Papers
-
Papers and patents are becoming less disruptive over time (Park et al., Nature 2023 cover 장식)
- News
-
OpenAI 지분 공개 매각 시도, 약 300억 달러 규모
- ChatGPT의 열풍과 더불어 지분 매각을 시도하는데, OpenAI의 가치가 높게 산정되고 있음
- OpenAI는 23년 목표 매출 2억 달러, 24년에는 10억 달러를 달성하겠다고 작년 말에 발표
- 23년에는 어떤 기능과 성능을 가진 모델을 만들어낼지?
-
Generative AI 기업에 22년에만 13억 7천만 달러의 투자가 유치됨
- 22년 한 해 동안 생성모델을 연구개발하는 회사에 대한 투자액만 13억 7천만 달러, 지난 5년간의 누적 투자액 총 규모와 비슷
- 여러 테크 분야의 스타트업에 대한 투자가 줄어들고 있지만, 내년에도 Generative AI 분야에 대한 투자는 지속될 것으로 전망
- 스타트업 투자의 현황도, 연구의 흐름도, 유저들의 반응도 생성 AI가 가장 뜨거운 감자인 듯합니다.
-
AI가 생성한 이미지, 텍스트 등의 데이터가 온라인에 돌아다니면 모델의 학습에 다시 들어갈 위험이 존재
- 신중한 데이터 수집과 검수가 필요할 것으로 보이지만..
- 네이버는 어떻게 하시나요?(질문)
-
- Diffusion Models already have a Semantic Latent Space
- https://arxiv.org/abs/2210.10960
- https://kwonminki.github.io/Asyrp/
- 제 논문 소개입니다...ㅎ
- Diffusion models의 bottleneck을 semantic latent space로 쓸 수 있다는 논문입니다
아직은 비공개인 링크도 살짝 올려보자면 (아직 arxiving 되지 않은 후속논문의 프로젝트페이지입니다.)
styletransfer 스러운 무언가도 할 수 있습니다.
https://curryjung.github.io/DiffStyle_project_page/
News
Conferences
- AAAI 2023 모두들 축하드립니다 (뒷북)
- ACL 2023: Softconf 1월 17일
- ICML 2023: Openreview 1월 26일 (한국 시간 27일 새벽 5시.. )
- CVPR 2023: 리뷰 1월 10일까지.. Aㅏ.....
Anthropic 의 Claude 가 ChatGPT3 보다 낫다는데...
- https://twitter.com/goodside/status/1611456645737107456?s=20&t=mT035MdDp9zDpowONheydg
- Constitutional AI: Harmlessness from AI Feedback
![]()
Research
Neural Codec Language Models are Zero-Shot Text to Speech Synthesizers
- MS에서 공개된 DALL-E 스타일의 Zero-shot Text-to-speech version. (음성 스타일 + 화자)
- Of-the-shelf audio encoder --> VQ token --> Neural Codec LM --> Conditional LM token 생성 --> Audio decoder
- Text prompt --> Phoneme conversion token, Audio prompt --> audio codec encoder token
- 프로젝트 & 데모: https://valle-demo.github.io/
![]()
![]()
Muse: Text-To-Image Generation via Masked Generative Transformers
- Google에서 나온 Transformer 기반 Masked Image Model 스타일 Text-to-image generation
- VQ token 레벨의 Masking. Text encoder (T5-XXL), Base MIM Transformer, HR Superresolution Transformer,
- 기존 Pixel level diffusion (Imagen, DALLE2) 나 Parti (autoregressive) 보다 이래 저래 효율적이라는..
- 물론 VQ tokenizer는 필수. Masking ratio는 Cosine scheduling (MaskGIT)
![]()
![]()
![]()
![]()
흥미있는 연구
Large Language Models as Corporate Lobbyists
- 제목 그대로.. 대기업의 B2B SaaS 상품화??
Scalable Diffusion Models with Transformers: LDM의 Attention UNet 을 Transformer로
Cool Japan Animation by FT Stable Diffusion: 당신이 애니덕후라면..
언제나 좋은 정보 공유 감사드려요~ 새해 복 많이 받으소서!