dqc
dqc copied to clipboard
Add initial asv benchmarks
This PR begins work towards #12 by adding an initial airspeed velocity compatible benchmark. airspeed velocity must be installed and configured to run them.
Right now it includes just one benchmark file that has benchmarks for both dqc and PySCF. These could be split into two files if preferred.
I also just include a couple simple energy calculations for two molecules, based this notebook.
In my mind this PR is really just illustrative to give a sense of what might be possible. We can either continue discussion on what we want to include in #12 or here.
Here are the results of benchmarks on my 2019 macbook pro
(chem) laptop:dqc nsofroniew$ asv run -E existing -b TimePySCF
· virtualenv package not installed
· Discovering benchmarks
· Running 2 total benchmarks (1 commits * 1 environments * 2 benchmarks)
[ 0.00%] ·· Benchmarking existing-py_Users_nsofroniew_opt_anaconda3_envs_chem_bin_python
[ 25.00%] ··· Running (benchmarks.TimePySCF.time_energy_HF--)..
[ 75.00%] ··· benchmarks.TimePySCF.time_energy_HF ok
[ 75.00%] ··· ========== ============
molecule
---------- ------------
H2O 61.2±2ms
C4H5N 1.52±0.01s
========== ============
[100.00%] ··· benchmarks.TimePySCF.time_energy_LDA ok
[100.00%] ··· ========== ============
molecule
---------- ------------
H2O 316±3ms
C4H5N 4.78±0.02s
========== ============
(chem) laptop:dqc:dqc nsofroniew$ asv run -E existing -b TimeDQC
· virtualenv package not installed
· Discovering benchmarks
· Running 2 total benchmarks (1 commits * 1 environments * 2 benchmarks)
[ 0.00%] ·· Benchmarking existing-py_Users_nsofroniew_opt_anaconda3_envs_chem_bin_python
[ 25.00%] ··· Running (benchmarks.TimeDQC.time_energy_HF--).
[ 50.00%] ··· Running (benchmarks.TimeDQC.time_energy_LDA--).
[ 75.00%] ··· benchmarks.TimeDQC.time_energy_HF ok
[ 75.00%] ··· ========== ===========
molecule
---------- -----------
H2O 63.4±1ms
C4H5N 16.0±0.3s
========== ===========
[100.00%] ··· benchmarks.TimeDQC.time_energy_LDA ok
[100.00%] ··· ========== ============
molecule
---------- ------------
H2O 414±4ms
C4H5N 19.9±0.08s
========== ============
Codecov Report
Merging #13 (e152e75) into master (0fe821f) will decrease coverage by
0.68%
. The diff coverage is0.00%
.
@@ Coverage Diff @@
## master #13 +/- ##
==========================================
- Coverage 92.09% 91.40% -0.69%
==========================================
Files 72 73 +1
Lines 7564 7621 +57
==========================================
Hits 6966 6966
- Misses 598 655 +57
Flag | Coverage Δ | |
---|---|---|
unittests | 91.40% <0.00%> (-0.69%) |
:arrow_down: |
Flags with carried forward coverage won't be shown. Click here to find out more.
Impacted Files | Coverage Δ | |
---|---|---|
benchmarks/benchmarks.py | 0.00% <0.00%> (ø) |
Continue to review full report at Codecov.
Legend - Click here to learn more
Δ = absolute <relative> (impact)
,ø = not affected
,? = missing data
Powered by Codecov. Last update 0fe821f...e152e75. Read the comment docs.
I have now update this PR to include an additional benchmark_properties.py
. In this one I parameterize over the system and measure the time for calculation after a system has been calculated. I've only included dqc property calculations in this benchmark
We could do a similar multiparameter calculation for the original benchmark.py
if we wanted to as well. As I said above there are many different ways to structure the benchmarks and this is meant really only to give some illustrative examples
(chem) laptop:dqc nsofroniew$ asv run -E existing -b TimeProperties -q
· virtualenv package not installed
· Discovering benchmarks
· Running 3 total benchmarks (1 commits * 1 environments * 3 benchmarks)
[ 0.00%] ·· Benchmarking existing-py_Users_nsofroniew_opt_anaconda3_envs_chem_bin_python
[ 16.67%] ··· benchmark_properties.TimeProperties.time_energy ok
[ 16.67%] ··· ========== ========= ==========
-- system
---------- --------------------
molecule HF LDA
========== ========= ==========
H2O 737±0μs 12.9±0ms
C4H5N 106±0ms 230±0ms
========== ========= ==========
[ 33.33%] ··· benchmark_properties.TimeProperties.time_ir_spectrum ok
[ 33.33%] ··· ========== ========== =========
-- system
---------- --------------------
molecule HF LDA
========== ========== =========
H2O 14.2±0ms 447±0ms
C4H5N 1.33±0s 3.90±0s
========== ========== =========
[ 50.00%] ··· benchmark_properties.TimeProperties.time_vibration ok
[ 50.00%] ··· ========== ========== ==========
-- system
---------- ---------------------
molecule HF LDA
========== ========== ==========
H2O 4.48±0ms 16.7±0ms
C4H5N 114±0ms 231±0ms
========== ========== ==========
I have now refactored the benchmarks back into a single file with three different timing suites
- one for qccalcs in dqc
TimeCalcDQC
- one for qccalcs in PySCF
TimeCalcPySCF
- one for properties calculations in dqc
TimePropertiesDQC
I think it's a little cleaner in a single file and the results are easier to parse compared to the earlier commits.
Something for you to consider if you like not including the time for the qccalcs in the properties calculations in TimePropertiesDQC? (i.e. the
runcall is now in the setup file which is not include in the time). I like it because we're calculating that explicitly in
TimeCalcDQC`.
If you like the overall structure of the benchmarks then some other things to consider are do we want to include more qccalcs
, I know you mentioned direct & density fitting, so that would then become 4 instead of 2.
Some other potential things include
- other bases
- more molecules
- more properties
Looking forward to hearing what else, if anything you might like. Also let me know if you have problems running the benchmarks, some helpful commands are
asv run -E existing -b TimeCalcDQC -q
which will run the TimeCalcDQC
benchmark in your existing environment quickly -q
(i.e. just a single pass to check it all runs ok)
If you're curious you can also do some profiling with asv and vizualize with snakeviz as follows
asv profile "benchmarks.TimeCalcDQC.time_qccalc\('C4H5N', 'HF'\)" -g snakeviz --python=same
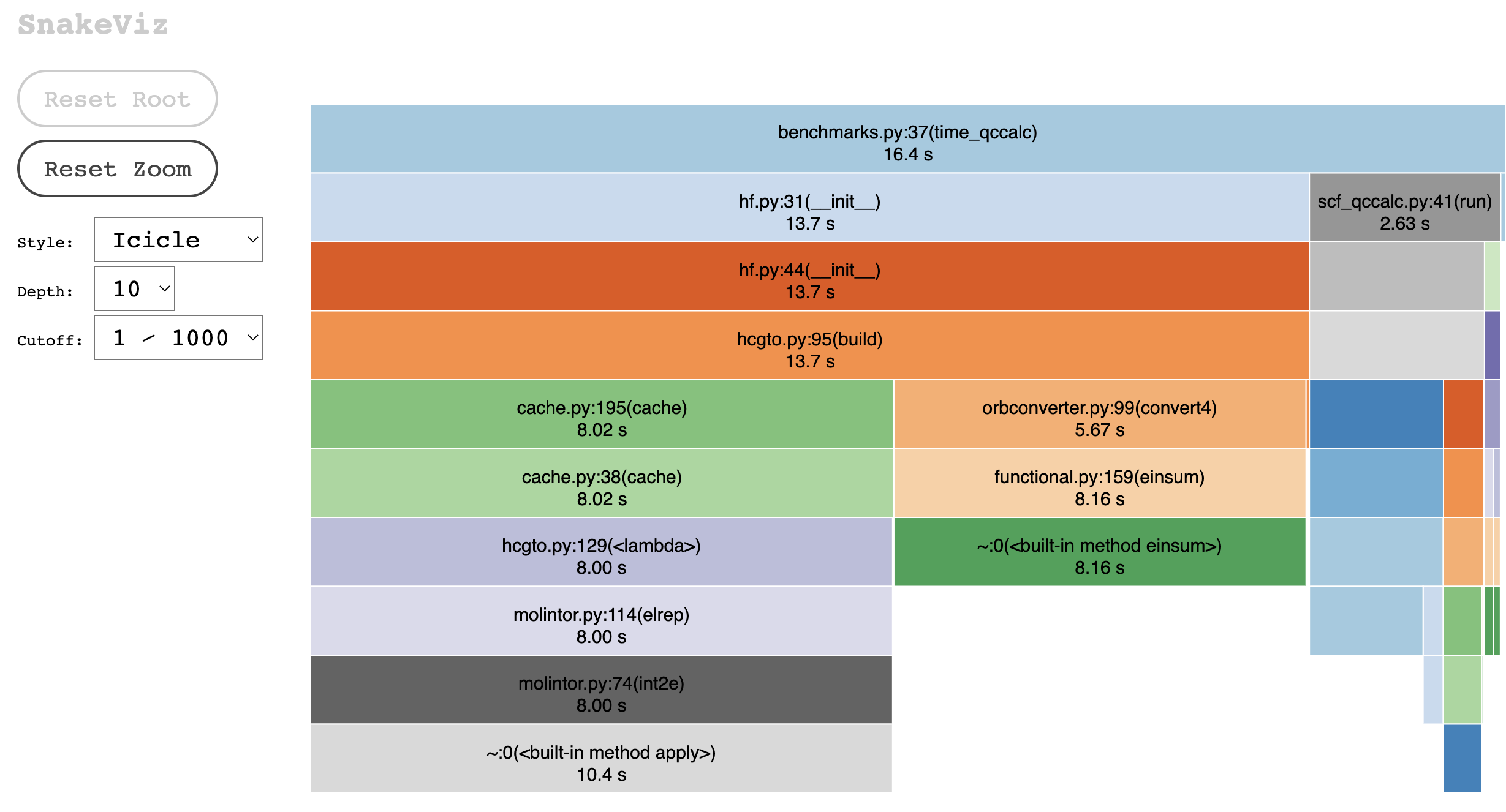
This is quite interesting as it looks like a lot of time is spent making calls to this function
https://github.com/diffqc/dqc/blob/0fe821fc92cb3457fb14f6dff0c223641c514ddb/dqc/hamilton/orbconverter.py#L99-L107
which would likely be faster on a gpu :-)
Anyway, just fun to play with! If you find the asv docs to detailed I wrote a simple asv benchmarking guide for a project where I am a maintainer, and it's got info on the commands that at least I tend to use the most
Nice! Thank you very much for the benchmarking. Would you mind to add a README.md file in the benchmarks
directory a short instruction (+ maybe link to the napari page), so we wouldn't have to do the search to run the benchmark.
To include the 1 iteration SCF benchmark, in DQC you can do something like qc.run(fwd_options={"maxiter": 1})
(it is undocumented, sorry).