ChatGLM-6B
ChatGLM-6B copied to clipboard
建议可以流式输出
类似chatGPT那样,一次输出一句话或者一个字,这样当机器人回复文字过长时,不需要那么长的等待时间。
臣附议
附议,这样才算chat
+1
chatgpt那个只是前端效果吧。
chatgpt那个只是前端效果吧。
print或者网页输出的时候,正常情况下会有缓存区,满了之后或者内容完整后一次性输出,但是通过sse或者stream的方式实现逐字快速输出,我用openai的whisper实现过。
看起来如果需要改的话得从hf hub里面的modeling_chatglm.py中的generate函数开始
看起来如果需要改的话得从hf hub里面的modeling_chatglm.py中的generate函数开始
是的,改下逻辑,然后return改成yield
请问有示例代码吗?
我自己有一个办法来实现流式输出,但是比较愚蠢,想玩的可以参考一下,真正要做到能用可能还是得参考一下现有的一些流式输出的项目。下面是代码参考:
import os
import platform
from transformers import AutoTokenizer, AutoModel
tokenizer = AutoTokenizer.from_pretrained("THUDM/chatglm-6b", trust_remote_code=True)
model = AutoModel.from_pretrained("THUDM/chatglm-6b", trust_remote_code=True).half().cuda()
model = model.eval()
os_name = platform.system()
first_out = True
decode_cache = []
def output_highjack(*args,**kwargs):
global first_out,decode_cache
res = _prepare_inputs_for_generation(*args,**kwargs)
if first_out:
print("ChatGLM-6B:",end="")
else:
id = res["input_ids"][-1]
decode_cache.append(id)
out = tokenizer.decode(decode_cache)
if "�" not in out:
print(out,end="")
decode_cache = []
first_out=False
return res
_prepare_inputs_for_generation=model.prepare_inputs_for_generation
model.prepare_inputs_for_generation=output_highjack
history = []
print("欢迎使用 ChatGLM-6B 模型,输入内容即可进行对话,clear 清空对话历史,stop 终止程序")
while True:
query = input("\n用户:")
if query == "stop":
break
if query == "clear":
history = []
command = 'cls' if os_name == 'Windows' else 'clear'
os.system(command)
print("欢迎使用 ChatGLM-6B 模型,输入内容即可进行对话,clear 清空对话历史,stop 终止程序")
continue
response, history = model.chat(tokenizer, query, history=history,do_sample=True)
first_out = True
我也有一个实现流式输出的方法,通过控制generate()
的max_length
实现
修改modeling_chatglm.py
文件,在ChatGLMForConditionalGeneration
类新增两个函数
@torch.no_grad()
def chat_stream(self, tokenizer, query: str, history: List[Tuple[str, str]] = None, delta_tokens: int = 2, max_length: int = 2048,
num_beams=1, do_sample=True, top_p=0.7, temperature=0.95, logits_processor=None, **kwargs):
if history is None:
history = []
if logits_processor is None:
logits_processor = LogitsProcessorList()
logits_processor.append(InvalidScoreLogitsProcessor())
gen_kwargs = {"num_beams": num_beams, "do_sample": do_sample, "top_p": top_p, "temperature": temperature,
"eos_token_id": 150005, "logits_processor": logits_processor, **kwargs}
if not history:
prompt = query
else:
prompt = ""
for i, (old_query, response) in enumerate(history):
prompt += "[Round {}]\n问:{}\n答:{}\n".format(i, old_query, response)
prompt += "[Round {}]\n问:{}\n答:".format(len(history), query)
input_ids = tokenizer([prompt], return_tensors="pt", padding=True)
input_ids = input_ids.to(self.device)
for delta, seq in self.generate_stream(delta_tokens, max_length, **input_ids, **gen_kwargs):
if delta:
delta = tokenizer.decode(delta)
else:
delta = ""
if seq:
seq = tokenizer.decode(seq)
else:
seq = ""
yield delta, seq, history + [(query, seq)]
@torch.no_grad()
def generate_stream(self, delta_tokens, max_length, **kwargs):
eos = kwargs["eos_token_id"]
base_p = len(kwargs["input_ids"][0])
last_p = base_p
kwargs["max_length"] = len(kwargs["input_ids"][0]) + delta_tokens
while True:
output_ids = super().generate(**kwargs)
output_seq = output_ids[0].tolist()
if eos in output_seq:
eos_p = output_seq.index(eos)
else:
eos_p = len(output_seq)
return_delta = output_seq[last_p: eos_p]
return_seq = output_seq[base_p: eos_p]
yield return_delta, return_seq
if eos in output_seq or kwargs["max_length"] >= max_length:
break
kwargs["input_ids"] = output_ids
kwargs["max_length"] = len(output_ids[0]) + delta_tokens
last_p = eos_p
chat_stream()
是个生成器函数,可以通for循环逐步输出
for delta, seq, history in model.chat_stream(tokenizer, query, history=history, delta_tokens=2):
print(delta, end="")
delta
为每次的增量输出,seq
为累计输出。参数 delta_tokens
控制每次增量输出的token数量
完整的调用demo:
import os, sys
import platform
from transformers import AutoTokenizer, AutoModel
tokenizer = AutoTokenizer.from_pretrained("chatglm-6b", trust_remote_code=True)
model = AutoModel.from_pretrained("chatglm-6b", trust_remote_code=True).half().quantize(4).cuda()
model = model.eval()
os_name = platform.system()
history = []
print("欢迎使用 ChatGLM-6B 模型,输入内容即可进行对话,clear 清空对话历史,stop 终止程序")
while True:
query = input("\n用户:")
if query == "stop":
break
if query == "clear":
history = []
command = 'cls' if os_name == 'Windows' else 'clear'
os.system(command)
print("欢迎使用 ChatGLM-6B 模型,输入内容即可进行对话,clear 清空对话历史,stop 终止程序")
continue
print(f"ChatGLM-6B:", end="")
for delta, seq, history in model.chat_stream(tokenizer, query, history=history):
print(delta, end="")
sys.stdout.flush()
为了方便修改modeling_chatglm.py
文件,建议克隆hugging face的代码到工作目录,from_pretrained
方法改为从本地加载
感谢大家对本仓库的支持。目前已经增加了流式输出接口stream_chat
,并且更新了命令行和网页版的demo。欢迎大家试用。
修改样式为对话模式
from transformers import AutoModel, AutoTokenizer
import gradio as gr
tokenizer = AutoTokenizer.from_pretrained("chatglm_6b", trust_remote_code=True)
model = AutoModel.from_pretrained("chatglm_6b", trust_remote_code=True).half().cuda()
model = model.eval()
MAX_TURNS = 20
MAX_BOXES = MAX_TURNS * 2
def predict(input, max_length, top_p, temperature, history=None):
if history is None:
history = []
for response, history in model.stream_chat(tokenizer, input, history, max_length=max_length, top_p=top_p,
temperature=temperature):
updates = []
for query, response in history:
updates.append(gr.update(visible=True, value="🧑:" + query))
updates.append(gr.update(visible=True, value="🤖:" + response))
if len(updates) < MAX_BOXES:
updates = updates + [gr.Textbox.update(visible=False)] * (MAX_BOXES - len(updates))
yield [history] + updates
#chatbot {height: 400px; overflow: auto;}
#col_container {width: 700px; margin-left: auto; margin-right: auto;}
title = """
<h1 align="center">🤖Chatbot</h1>
<link rel="stylesheet" href="/path/to/styles/default.min.css">
<script src="/path/to/highlight.min.js"></script>
<script>hljs.highlightAll();</script>
"""
css = """
#user {
float: left;
position:relative;
right:5px;
width:auto;
min-height:32px;
max-width: 60%
line-height: 32px;
padding: 2px 8px;
font-size: 14px;
background: #77FF00;
border-radius:5px; /* 圆角 */
margin:10px 0px;
}
#chatbot {
float: right;
position:relative;
right:5px;
width:auto;
min-height:32px;
max-width: 60%
line-height: 32px;
padding: 2px 8px;
font-size: 14px;
background:#F8C301;
border-radius:5px; /* 圆角 */
margin:10px 0px;
}
"""
with gr.Blocks(css=css) as demo:
gr.HTML(title)
state = gr.State([])
text_boxes = []
for i in range(MAX_BOXES):
if i % 2 == 0:
text_boxes.append(gr.Markdown(visible=False, label="提问:", elem_id="user"))
else:
text_boxes.append(gr.Markdown(visible=False, label="回复:", elem_id="chatbot"))
with gr.Column(elem_id = "col_container"):
with gr.Row():
with gr.Column(scale=19):
txt = gr.Textbox(
show_label=False,
placeholder="Enter text and press enter",
).style(container=True)
with gr.Column(scale=1):
button = gr.Button("Send")
with gr.Accordion("Parameters", open=False):
max_length = gr.Slider(0, 4096, value=2048, step=1.0, label="Maximum length", interactive=True)
top_p = gr.Slider(0, 1, value=0.7, step=0.01, label="Top P", interactive=True)
temperature = gr.Slider(0, 1, value=0.95, step=0.01, label="Temperature", interactive=True)
button.click(predict, [txt, max_length, top_p, temperature, state], [state] + text_boxes)
demo.queue().launch(share=False, inbrowser=True)
感谢大家对本仓库的支持。目前已经增加了流式输出接口
stream_chat
,并且更新了命令行和网页版的demo。欢迎大家试用。
目前的这个stream_chat的实现,严格意义上应该不能叫流式输出,而是一种类似于early access式的实现,每次返回都是把当前的完整输出给返回的,而不是返回上次输出后新增的内容。
我想问这样做是有意这样去设计的吗?除非像语音识别那样,后期的结果可能会修改早期返回的结果,不然我觉得这样做没什么好处。可以看到目前的cli_demo.py的实现,其实都是每次返回后要清空屏幕输出再显示内容,如果机器速度慢一点是肉眼可见的闪烁感。web_demo.py也类似。
如果不会修改早期返回的结果,我们完全可以自行修改成标准的流式实现吧,尤其是websocket的效果,代码会大大简化。
请问一下,chat或者stream_chat中调用的generate函数是如何判断要停止生成的呢?看了一下源码好像是没有特殊的限制的话,只会到达max_length或者max_time的时候才会停止。是通过什么其他的标准,比如eos或者换行符来判断的吗?
你的邮箱我已收到,谢谢! 唐湘润
from transformers import AutoModel, AutoTokenizer import gradio as gr tokenizer = AutoTokenizer.from_pretrained("chatglm_6b", trust_remote_code=True) model = AutoModel.from_pretrained("chatglm_6b", trust_remote_code=True).half().cuda() model = model.eval() MAX_TURNS = 20 MAX_BOXES = MAX_TURNS * 2 def predict(input, max_length, top_p, temperature, history=None): if history is None: history = [] for response, history in model.stream_chat(tokenizer, input, history, max_length=max_length, top_p=top_p, temperature=temperature): updates = [] for query, response in history: updates.append(gr.update(visible=True, value="🧑:" + query)) updates.append(gr.update(visible=True, value="🤖:" + response)) if len(updates) < MAX_BOXES: updates = updates + [gr.Textbox.update(visible=False)] * (MAX_BOXES - len(updates)) yield [history] + updates #chatbot {height: 400px; overflow: auto;} #col_container {width: 700px; margin-left: auto; margin-right: auto;} title = """ <h1 align="center">🤖Chatbot</h1> <link rel="stylesheet" href="/path/to/styles/default.min.css"> <script src="/path/to/highlight.min.js"></script> <script>hljs.highlightAll();</script> """ css = """ #user { float: left; position:relative; right:5px; width:auto; min-height:32px; max-width: 60% line-height: 32px; padding: 2px 8px; font-size: 14px; background: #77FF00; border-radius:5px; /* 圆角 */ margin:10px 0px; } #chatbot { float: right; position:relative; right:5px; width:auto; min-height:32px; max-width: 60% line-height: 32px; padding: 2px 8px; font-size: 14px; background:#F8C301; border-radius:5px; /* 圆角 */ margin:10px 0px; } """ with gr.Blocks(css=css) as demo: gr.HTML(title) state = gr.State([]) text_boxes = [] for i in range(MAX_BOXES): if i % 2 == 0: text_boxes.append(gr.Markdown(visible=False, label="提问:", elem_id="user")) else: text_boxes.append(gr.Markdown(visible=False, label="回复:", elem_id="chatbot")) with gr.Column(elem_id = "col_container"): with gr.Row(): with gr.Column(scale=19): txt = gr.Textbox( show_label=False, placeholder="Enter text and press enter", ).style(container=True) with gr.Column(scale=1): button = gr.Button("Send") with gr.Accordion("Parameters", open=False): max_length = gr.Slider(0, 4096, value=2048, step=1.0, label="Maximum length", interactive=True) top_p = gr.Slider(0, 1, value=0.7, step=0.01, label="Top P", interactive=True) temperature = gr.Slider(0, 1, value=0.95, step=0.01, label="Temperature", interactive=True) button.click(predict, [txt, max_length, top_p, temperature, state], [state] + text_boxes) demo.queue().launch(share=False, inbrowser=True)
请问流式的换行 在前端,是如何做到的,还是说这个换行在服务端处理完了
你的邮箱我已收到,谢谢! 唐湘润
[ 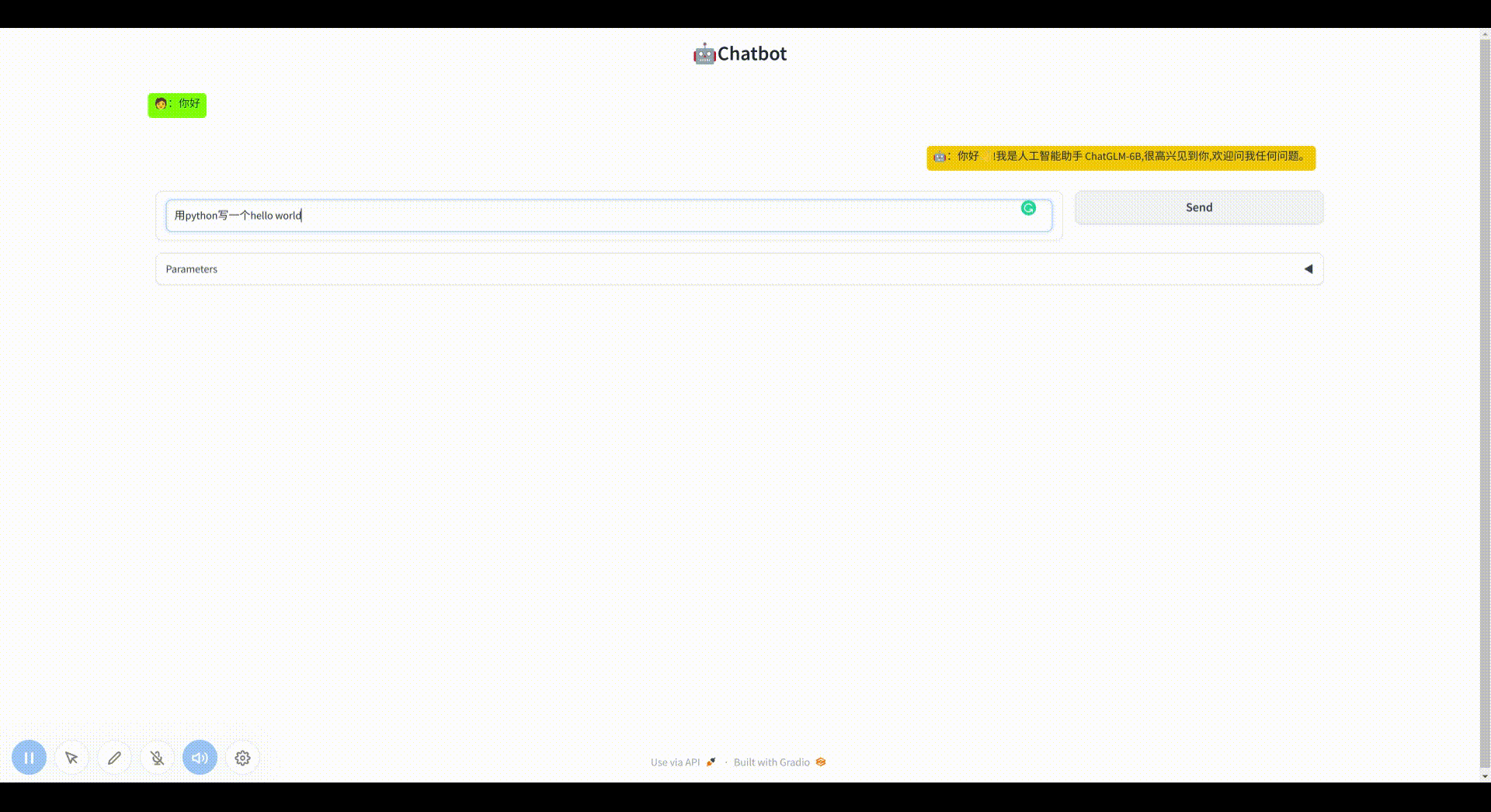 ](https://user-images.githubusercontent.com/28218658/226168560-8ce14040-9d3d-4384-a5f1-a7ae426cca46.gif) [ ](https://user-images.githubusercontent.com/28218658/226168560-8ce14040-9d3d-4384-a5f1-a7ae426cca46.gif) [ 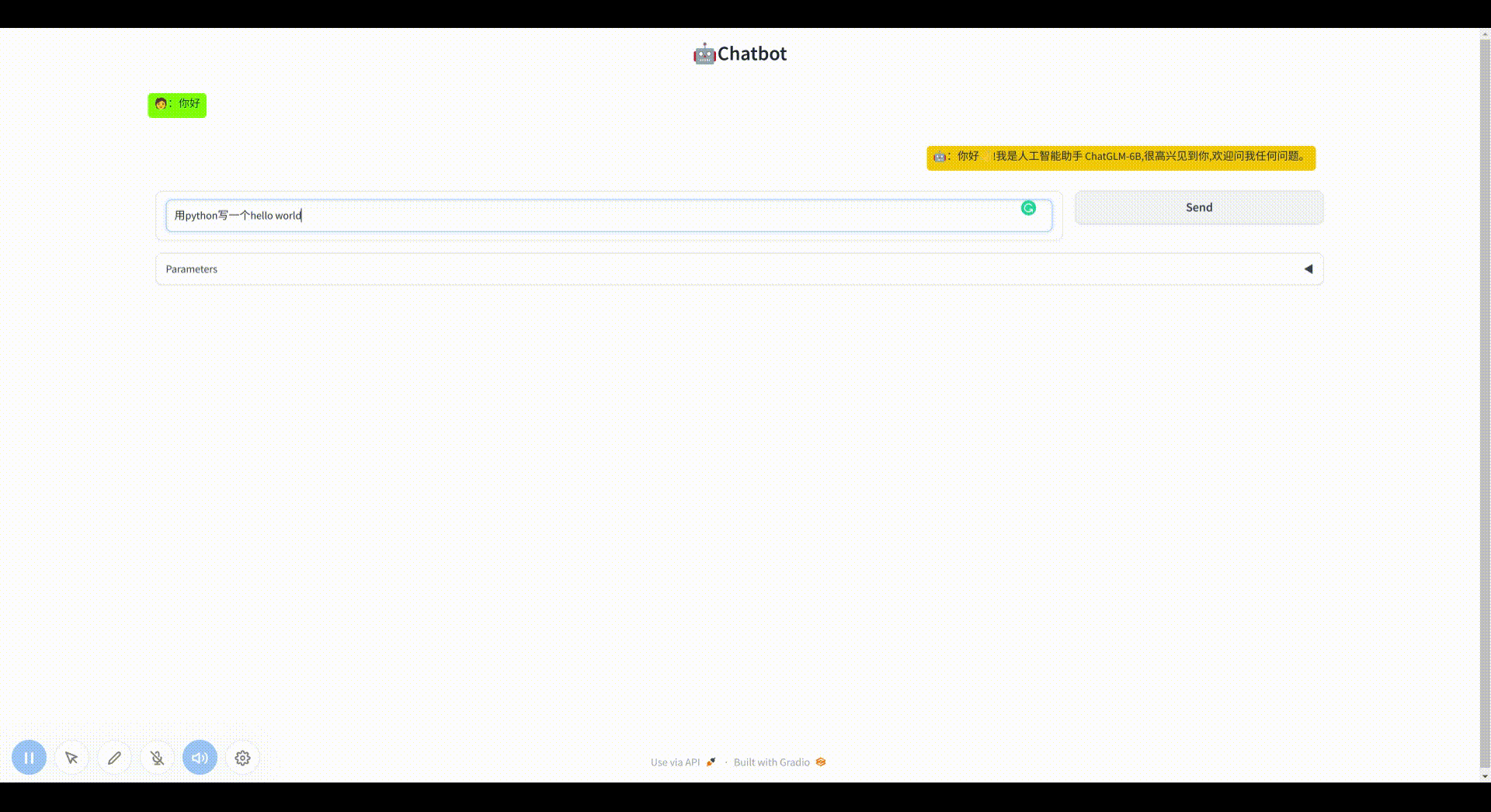 ](https://user-images.githubusercontent.com/28218658/226168560-8ce14040-9d3d-4384-a5f1-a7ae426cca46.gif) [ 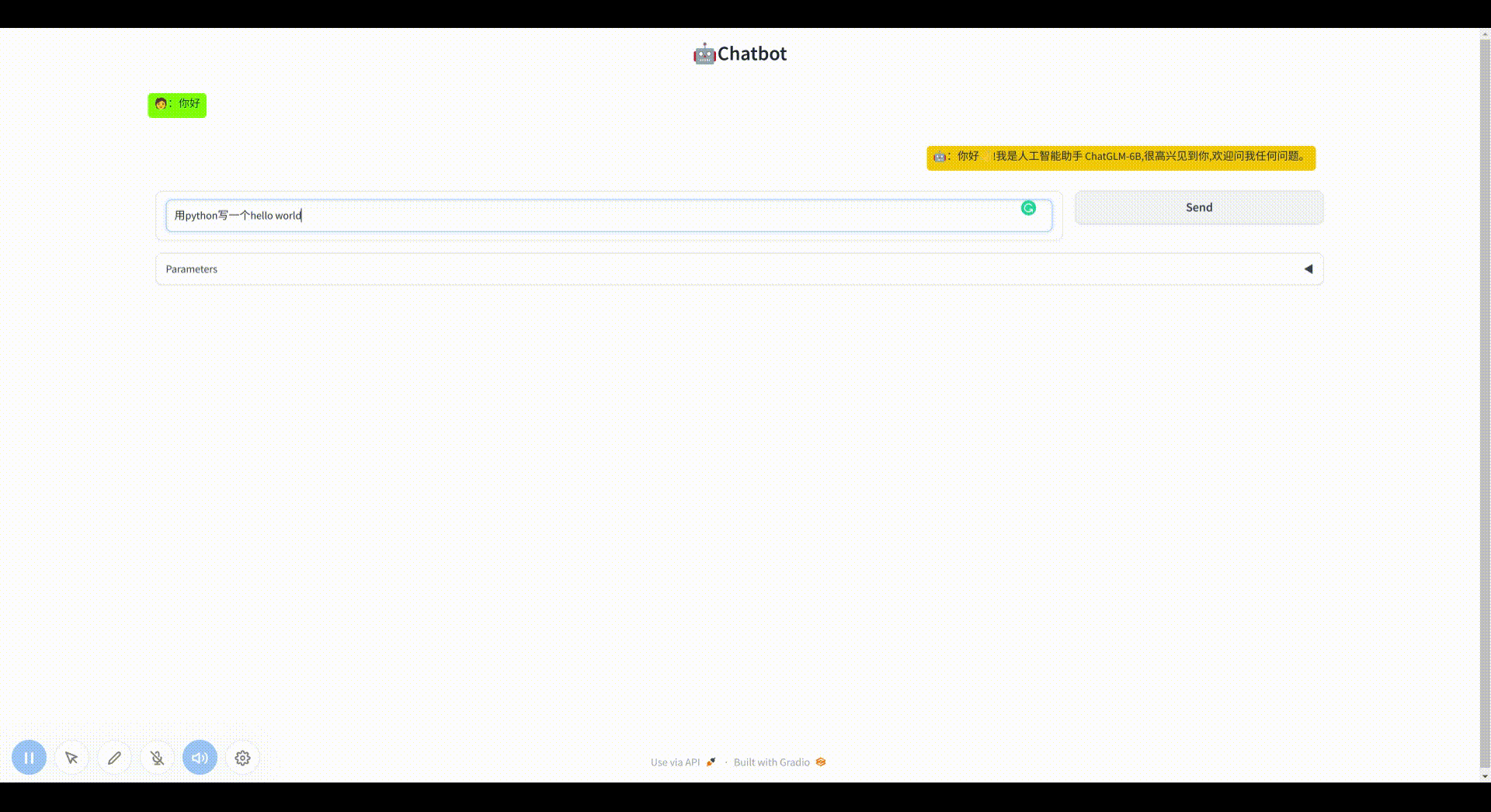 ](https://user-images.githubusercontent.com/28218658/226168560-8ce14040-9d3d-4384-a5f1-a7ae426cca46.gif) [ ](https://user-images.githubusercontent.com/28218658/226168560-8ce14040-9d3d-4384-a5f1-a7ae426cca46.gif) [ ](https://user-images.githubusercontent.com/28218658/226168560-8ce14040-9d3d-4384-a5f1-a7ae426cca46.gif) 修改样式为对话模式
from transformers import AutoModel, AutoTokenizer import gradio as gr tokenizer = AutoTokenizer.from_pretrained("chatglm_6b", trust_remote_code=True) model = AutoModel.from_pretrained("chatglm_6b", trust_remote_code=True).half().cuda() model = model.eval() MAX_TURNS = 20 MAX_BOXES = MAX_TURNS * 2 def predict(input, max_length, top_p, temperature, history=None): if history is None: history = [] for response, history in model.stream_chat(tokenizer, input, history, max_length=max_length, top_p=top_p, temperature=temperature): updates = [] for query, response in history: updates.append(gr.update(visible=True, value="🧑:" + query)) updates.append(gr.update(visible=True, value="🤖:" + response)) if len(updates) < MAX_BOXES: updates = updates + [gr.Textbox.update(visible=False)] * (MAX_BOXES - len(updates)) yield [history] + updates #chatbot {height: 400px; overflow: auto;} #col_container {width: 700px; margin-left: auto; margin-right: auto;} title = """ <h1 align="center">🤖Chatbot</h1> <link rel="stylesheet" href="/path/to/styles/default.min.css"> <script src="/path/to/highlight.min.js"></script> <script>hljs.highlightAll();</script> """ css = """ #user { float: left; position:relative; right:5px; width:auto; min-height:32px; max-width: 60% line-height: 32px; padding: 2px 8px; font-size: 14px; background: #77FF00; border-radius:5px; /* 圆角 */ margin:10px 0px; } #chatbot { float: right; position:relative; right:5px; width:auto; min-height:32px; max-width: 60% line-height: 32px; padding: 2px 8px; font-size: 14px; background:#F8C301; border-radius:5px; /* 圆角 */ margin:10px 0px; } """ with gr.Blocks(css=css) as demo: gr.HTML(title) state = gr.State([]) text_boxes = [] for i in range(MAX_BOXES): if i % 2 == 0: text_boxes.append(gr.Markdown(visible=False, label="提问:", elem_id="user")) else: text_boxes.append(gr.Markdown(visible=False, label="回复:", elem_id="chatbot")) with gr.Column(elem_id = "col_container"): with gr.Row(): with gr.Column(scale=19): txt = gr.Textbox( show_label=False, placeholder="Enter text and press enter", ).style(container=True) with gr.Column(scale=1): button = gr.Button("Send") with gr.Accordion("Parameters", open=False): max_length = gr.Slider(0, 4096, value=2048, step=1.0, label="Maximum length", interactive=True) top_p = gr.Slider(0, 1, value=0.7, step=0.01, label="Top P", interactive=True) temperature = gr.Slider(0, 1, value=0.95, step=0.01, label="Temperature", interactive=True) button.click(predict, [txt, max_length, top_p, temperature, state], [state] + text_boxes) demo.queue().launch(share=False, inbrowser=True)
请问流式的换行 在前端,是如何做到的,还是说这个换行在服务端处理完了
web自动处理,服务端会把累计文本发送给前端。比如 你 你好 你好世 你好世界
[ 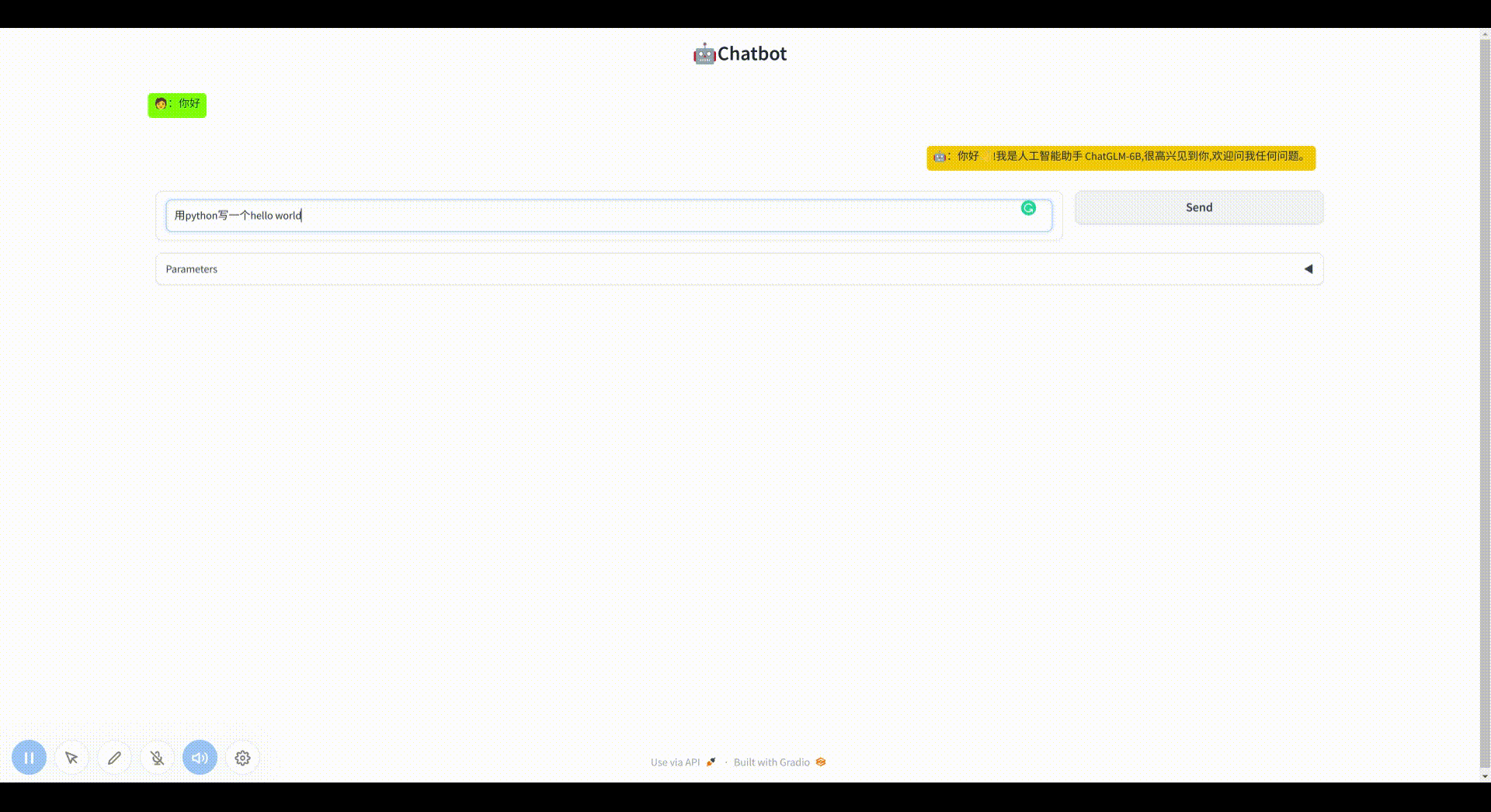 ](https://user-images.githubusercontent.com/28218658/226168560-8ce14040-9d3d-4384-a5f1-a7ae426cca46.gif) [ ](https://user-images.githubusercontent.com/28218658/226168560-8ce14040-9d3d-4384-a5f1-a7ae426cca46.gif)
[ 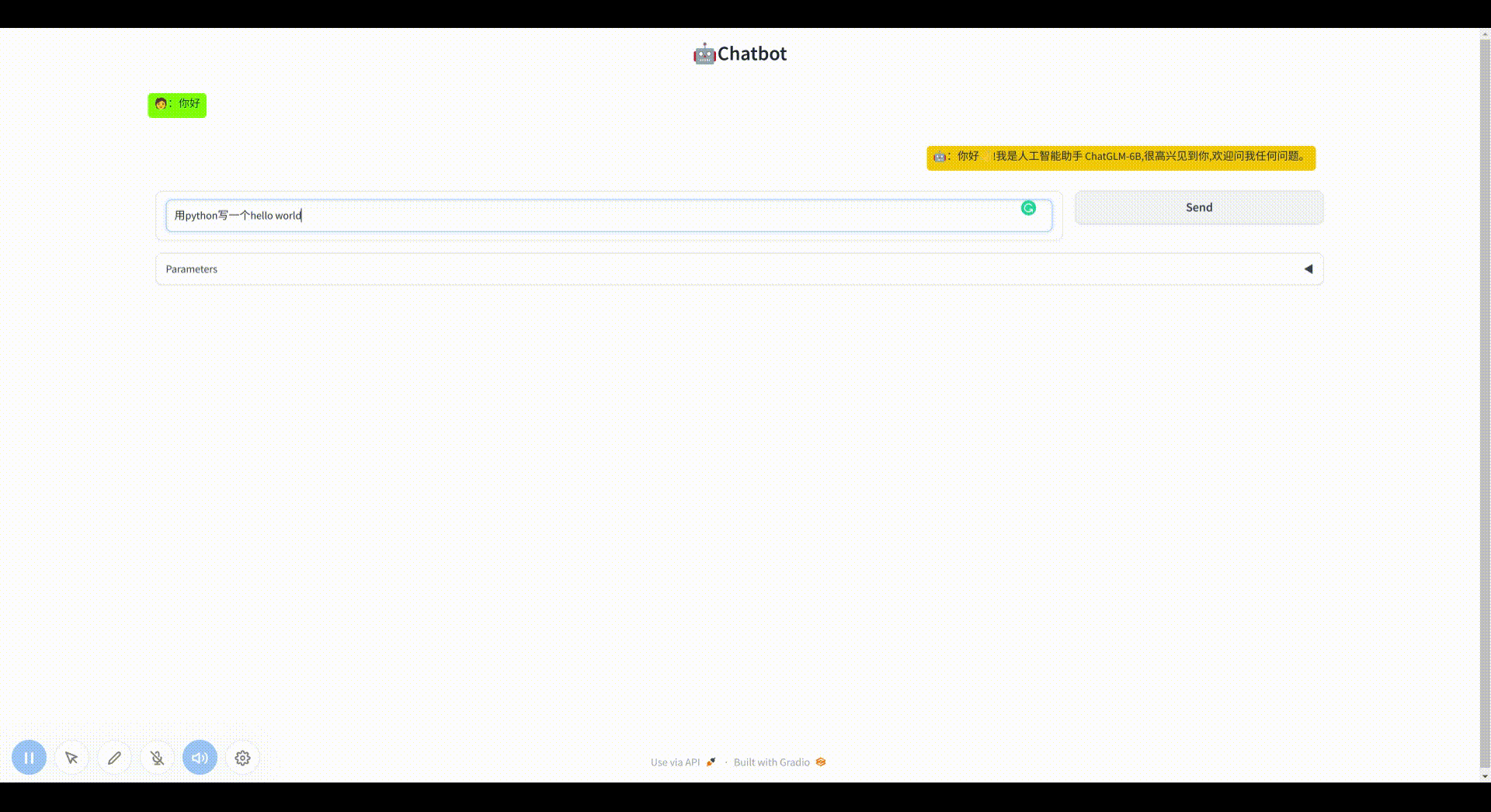 ](https://user-images.githubusercontent.com/28218658/226168560-8ce14040-9d3d-4384-a5f1-a7ae426cca46.gif) [ ](https://user-images.githubusercontent.com/28218658/226168560-8ce14040-9d3d-4384-a5f1-a7ae426cca46.gif) [ 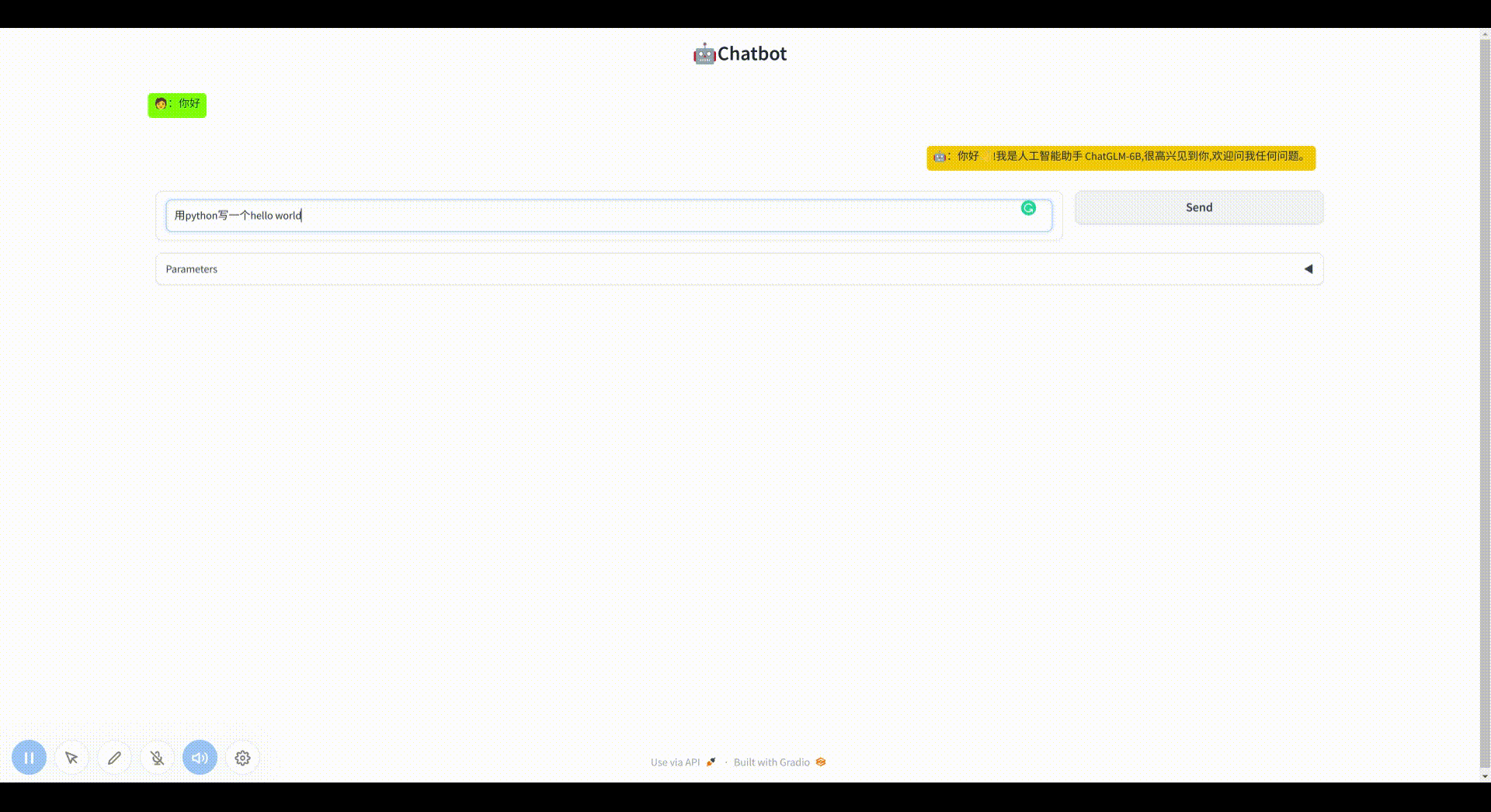 ](https://user-images.githubusercontent.com/28218658/226168560-8ce14040-9d3d-4384-a5f1-a7ae426cca46.gif) [ 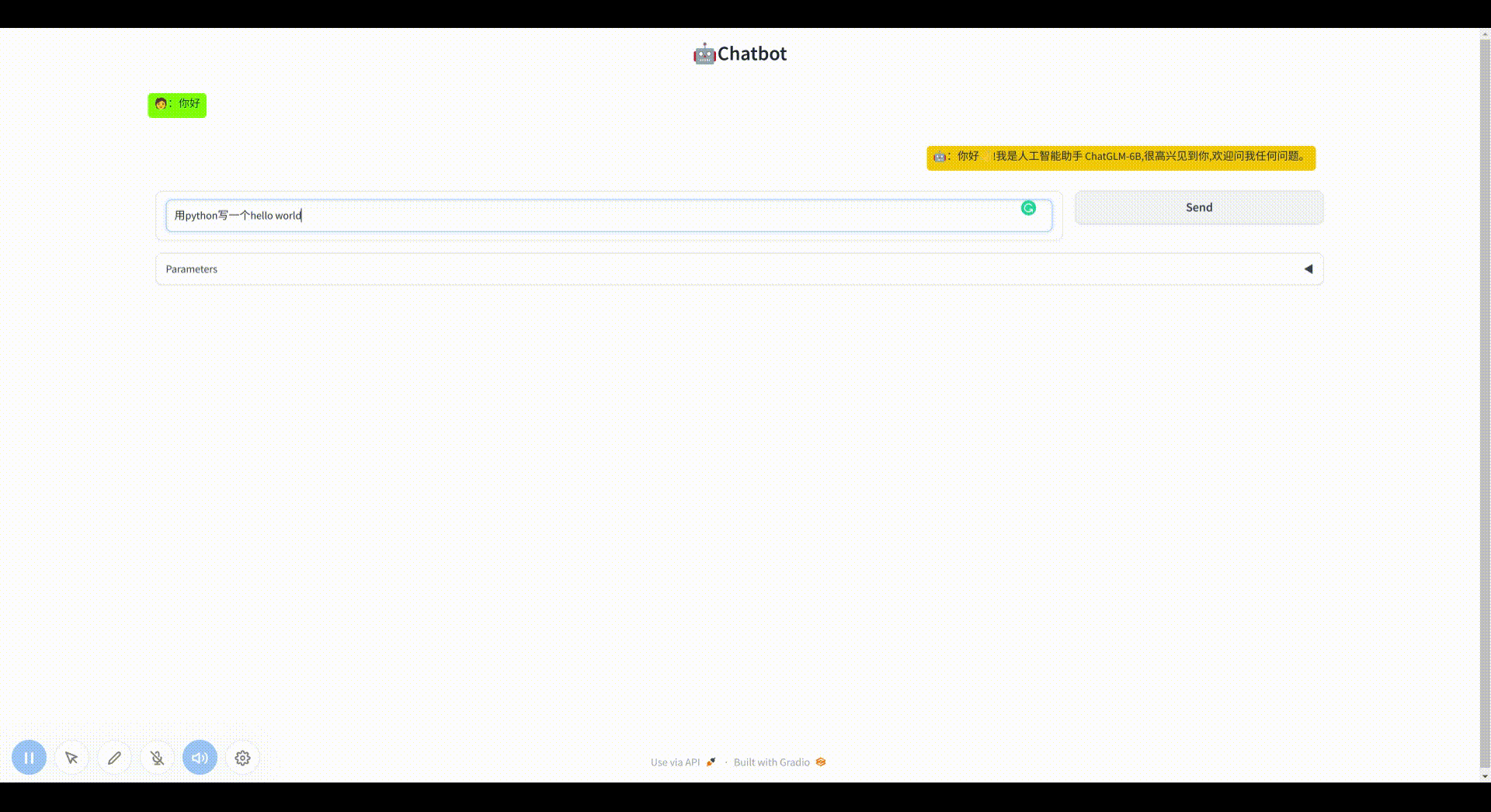 ](https://user-images.githubusercontent.com/28218658/226168560-8ce14040-9d3d-4384-a5f1-a7ae426cca46.gif) [ ](https://user-images.githubusercontent.com/28218658/226168560-8ce14040-9d3d-4384-a5f1-a7ae426cca46.gif) [ ](https://user-images.githubusercontent.com/28218658/226168560-8ce14040-9d3d-4384-a5f1-a7ae426cca46.gif) 修改样式为对话模式
from transformers import AutoModel, AutoTokenizer import gradio as gr tokenizer = AutoTokenizer.from_pretrained("chatglm_6b", trust_remote_code=True) model = AutoModel.from_pretrained("chatglm_6b", trust_remote_code=True).half().cuda() model = model.eval() MAX_TURNS = 20 MAX_BOXES = MAX_TURNS * 2 def predict(input, max_length, top_p, temperature, history=None): if history is None: history = [] for response, history in model.stream_chat(tokenizer, input, history, max_length=max_length, top_p=top_p, temperature=temperature): updates = [] for query, response in history: updates.append(gr.update(visible=True, value="🧑:" + query)) updates.append(gr.update(visible=True, value="🤖:" + response)) if len(updates) < MAX_BOXES: updates = updates + [gr.Textbox.update(visible=False)] * (MAX_BOXES - len(updates)) yield [history] + updates #chatbot {height: 400px; overflow: auto;} #col_container {width: 700px; margin-left: auto; margin-right: auto;} title = """ <h1 align="center">🤖Chatbot</h1> <link rel="stylesheet" href="/path/to/styles/default.min.css"> <script src="/path/to/highlight.min.js"></script> <script>hljs.highlightAll();</script> """ css = """ #user { float: left; position:relative; right:5px; width:auto; min-height:32px; max-width: 60% line-height: 32px; padding: 2px 8px; font-size: 14px; background: #77FF00; border-radius:5px; /* 圆角 */ margin:10px 0px; } #chatbot { float: right; position:relative; right:5px; width:auto; min-height:32px; max-width: 60% line-height: 32px; padding: 2px 8px; font-size: 14px; background:#F8C301; border-radius:5px; /* 圆角 */ margin:10px 0px; } """ with gr.Blocks(css=css) as demo: gr.HTML(title) state = gr.State([]) text_boxes = [] for i in range(MAX_BOXES): if i % 2 == 0: text_boxes.append(gr.Markdown(visible=False, label="提问:", elem_id="user")) else: text_boxes.append(gr.Markdown(visible=False, label="回复:", elem_id="chatbot")) with gr.Column(elem_id = "col_container"): with gr.Row(): with gr.Column(scale=19): txt = gr.Textbox( show_label=False, placeholder="Enter text and press enter", ).style(container=True) with gr.Column(scale=1): button = gr.Button("Send") with gr.Accordion("Parameters", open=False): max_length = gr.Slider(0, 4096, value=2048, step=1.0, label="Maximum length", interactive=True) top_p = gr.Slider(0, 1, value=0.7, step=0.01, label="Top P", interactive=True) temperature = gr.Slider(0, 1, value=0.95, step=0.01, label="Temperature", interactive=True) button.click(predict, [txt, max_length, top_p, temperature, state], [state] + text_boxes) demo.queue().launch(share=False, inbrowser=True)
请问流式的换行 在前端,是如何做到的,还是说这个换行在服务端处理完了
web自动处理,服务端会把累计文本发送给前端。比如 你 你好 你好世 你好世界
找到问题了换行被服务端吃掉了 哈哈
请问怎么让本地的chatglm2-6b结合langchain使用流式输出呢? 已经写好了一个把chatglm2-6b接入langchain的代码了。
為什麼我用langchain的rag架構之後無法使用到stream output,程式如下。是否有人有相關經驗在langchian rag並使用stream output呢? Thanks for your help 更新一下:如果load自己的模型就無法steam output,但是使用openai模型就可以在Langchain框架之下==
rag_prompt_custom = PromptTemplate.from_template(template)
rag_chain = ({"context": self.retriever | self.format_docs, "question": RunnablePassthrough()}
| rag_prompt_custom
| self.llm
| StrOutputParser())
#response = rag_chain.invoke(query_text)
response = ""
for chunk in rag_chain.stream(query_text):
response+=chunk
print(chunk, end="", flush=True)
你的邮箱我已收到,谢谢! 唐湘润