slimmable_networks
slimmable_networks copied to clipboard
Slimmable Networks, AutoSlim, and Beyond, ICLR 2019, and ICCV 2019
Slimmable Networks
An open source framework for slimmable training on tasks of ImageNet classification and COCO detection, which has enabled numerous projects. 1, 2, 3
1. Slimmable Neural Networks ICLR 2019 Paper | OpenReview | Detection | Model Zoo
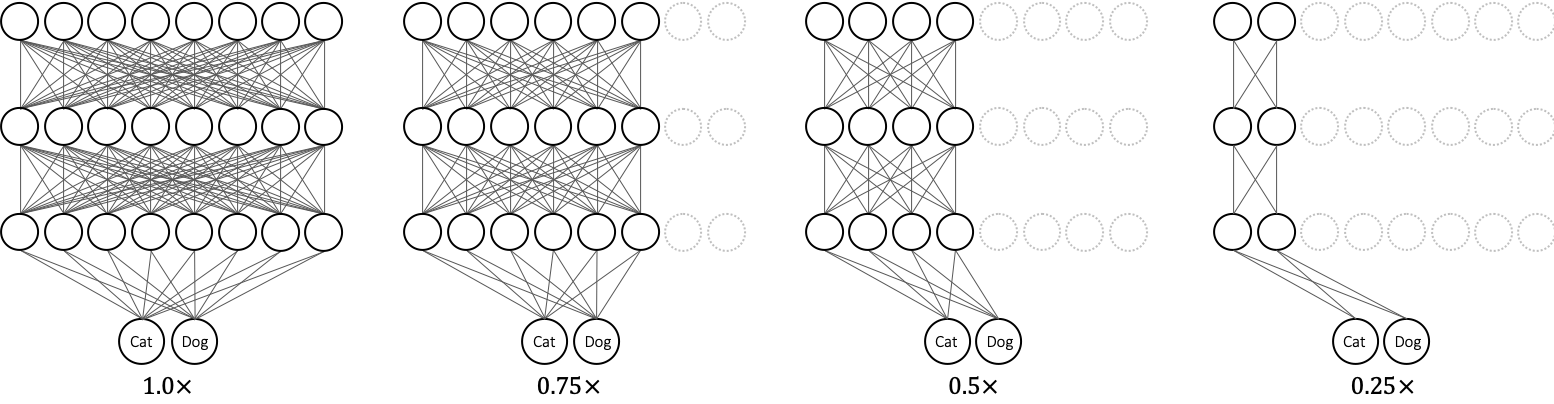
Illustration of slimmable neural networks. The same model can run at different widths (number of active channels), permitting instant and adaptive accuracy-efficiency trade-offs.
2. Universally Slimmable Networks and Improved Training Techniques ICCV 2019 Paper | Model Zoo
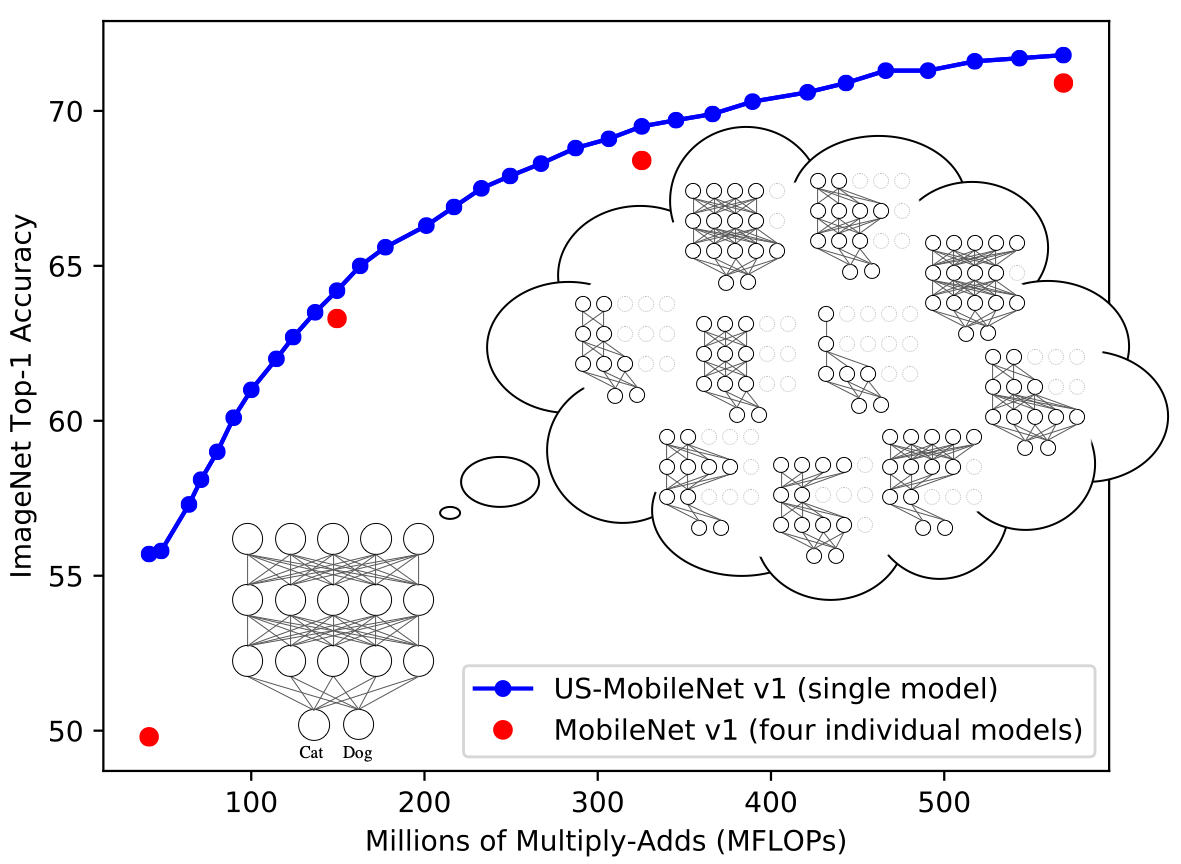
Illustration of universally slimmable networks. The same model can run at arbitrary widths.
3. AutoSlim: Towards One-Shot Architecture Search for Channel Numbers NeurIPS 2019 Workshop Paper | Model Zoo
AutoSlimming MobileNet v1, MobileNet v2, MNasNet and ResNet-50: the optimized number of channels under each computational budget (FLOPs).
Run
- Requirements:
- python3, pytorch 1.0, torchvision 0.2.1, pyyaml 3.13.
- Prepare ImageNet-1k data following pytorch example.
- Training and Testing:
- The codebase is a general ImageNet training framework using yaml config under
apps
dir, based on PyTorch. - To test, download pretrained models to
logs
dir and directly run command. - To train, comment
test_only
andpretrained
in config file. You will need to manage visible gpus by yourself. - Command:
python train.py app:{apps/***.yml}
.{apps/***.yml}
is config file. Do not missapp:
prefix. - Training and testing of MSCOCO benchmarks are released under branch detection.
- The codebase is a general ImageNet training framework using yaml config under
- Still have questions?
- If you still have questions, please search closed issues first. If the problem is not solved, please open a new.
Slimmable Model Zoo
Model | Switches (Widths) | Top-1 Err. | FLOPs | Model ID |
---|---|---|---|---|
S-MobileNet v1 | 1.00 0.75 0.50 0.25 |
28.5 30.5 35.2 46.9 |
569M 325M 150M 41M |
a6285db |
S-MobileNet v2 | 1.00 0.75 0.50 0.35 |
29.5 31.1 35.6 40.3 |
301M 209M 97M 59M |
0593ffd |
S-ShuffleNet | 2.00 1.00 0.50 |
28.6 34.5 42.8 |
524M 138M 38M |
1427f66 |
S-ResNet-50 | 1.00 0.75 0.50 0.25 |
24.0 25.1 27.9 35.0 |
4.1G 2.3G 1.1G 278M |
3fca9cc |
Universally Slimmable Networks and Improved Training Techniques
Model | Model ID | Spectrum | |||||||||||||||||||||||||||||||
---|---|---|---|---|---|---|---|---|---|---|---|---|---|---|---|---|---|---|---|---|---|---|---|---|---|---|---|---|---|---|---|---|---|
US‑MobileNet v1 | 13d5af2 | Width MFLOPs Top-1 Err. |
1.0 568 28.2 |
0.975 543 28.3 |
0.95 517 28.4 |
0.925 490 28.7 |
0.9 466 28.7 |
0.875 443 29.1 |
0.85 421 29.4 |
0.825 389 29.7 |
0.8 366 30.2 |
0.775 345 30.3 |
0.75 325 30.5 |
0.725 306 30.9 |
0.7 287 31.2 |
0.675 267 31.7 |
0.65 249 32.2 |
0.625 232 32.5 |
0.6 217 33.2 |
0.575 201 33.7 |
0.55 177 34.4 |
0.525 162 35.0 |
0.5 149 35.8 |
0.475 136 36.5 |
0.45 124 37.3 |
0.425 114 38.1 |
0.4 100 39.0 |
0.375 89 40.0 |
0.35 80 41.0 |
0.325 71 41.9 |
0.3 64 42.7 |
0.275 48 44.2 |
0.25 41 44.3 |
US‑MobileNet v2 | 3880cad | Width MFLOPs Top-1 Err. |
1.0 300 28.5 |
0.975 299 28.5 |
0.95 284 28.8 |
0.925 274 28.9 |
0.9 269 29.1 |
0.875 268 29.1 |
0.85 254 29.4 |
0.825 235 29.9 |
0.8 222 30.0 |
0.775 213 30.2 |
0.75 209 30.4 |
0.725 185 30.7 |
0.7 173 31.1 |
0.675 165 31.4 |
0.65 161 31.7 |
0.625 161 31.7 |
0.6 151 32.4 |
0.575 150 32.4 |
0.55 106 34.4 |
0.525 100 34.6 |
0.5 97 34.9 |
0.475 96 35.1 |
0.45 88 35.8 |
0.425 88 35.8 |
0.4 80 36.6 |
0.375 80 36.7 |
0.35 59 37.7 |
AutoSlim: Towards One-Shot Architecture Search for Channel Numbers
Model | Top-1 Err. | FLOPs | Model ID |
---|---|---|---|
AutoSlim-MobileNet v1 | 27.0 28.5 32.1 |
572M 325M 150M |
9b0b1ab |
AutoSlim-MobileNet v2 | 24.6 25.8 27.0 |
505M 305M 207M |
a24f1f2 |
AutoSlim-MNasNet | 24.6 25.4 26.8 |
532M 315M 217M |
31477c9 |
AutoSlim-ResNet-50 | 24.0 24.4 26.0 27.8 |
3.0G 2.0G 1.0G 570M |
f95f419 |
Technical Details
Implementing slimmable networks and slimmable training is straightforward:
- Switchable batchnorm and slimmable layers are implemented in
models/slimmable_ops
. - Slimmable training is implemented in these lines in
train.py
.
License
CC 4.0 Attribution-NonCommercial International
The software is for educaitonal and academic research purpose only.